The World of Data Science: Approaches
3AI October 9, 2020
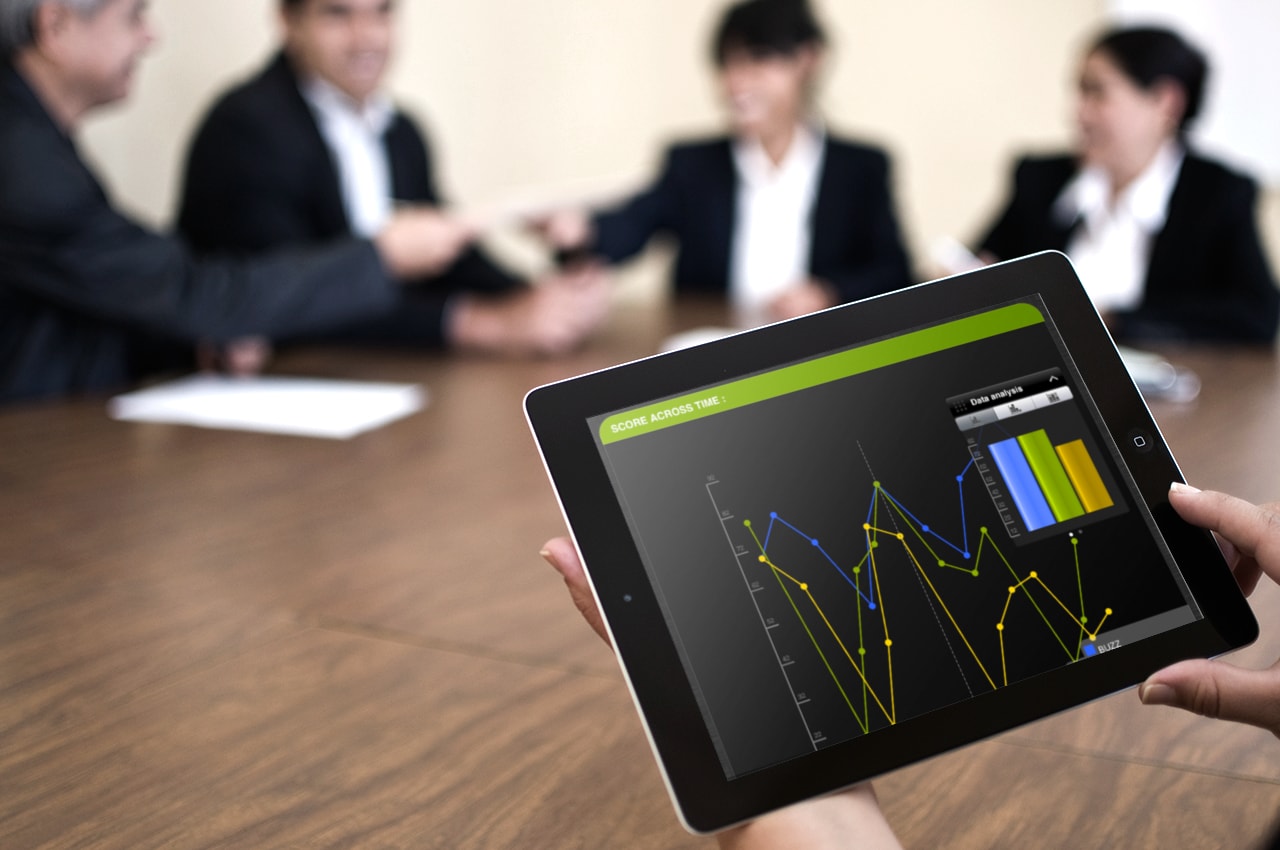
Unarguably, in today’s hyper-competitive marketplace, Data Science plays an indispensable role for organizations to personalize experiences and create value out of their data. Analyzing large data sets without preset defined rules or scope for analysis to uncover insights, a sublime concept till a few years ago, will form the key basis of competition in the future to significantly unlock business value, unleashing new waves of productivity for businesses, enabling a culture of innovation, and reinvigorating internal processes, as long as the right ecosystem and enablers are put in place.
Numerous articles today are buzzing with this glamourous new word in the Analytics world i.e. Data Science or Data Scientist. So what exactly is Data Science or this hype around Data Scientist?
Frankly speaking, multiple definitions, roles, job descriptions exist making it harder for businesses to understand what truly is the role about and the ROI out of making any additional investments. To me Data Science means mining actionable and sensible insights from the multiple data formats leveraging mathematics, statistics, machine learning etc. Data scientists typically analyze data sets, or data depositories that are maintained within an organization or scrape publically available data, and look at both upstream/downstream to enable business value transformation across the value chain. Data scientists are well-equipped with relevant statistical models and analyze voluminous past/current data stacks to derive recommendations and suggestions for optimal business decision making. Data scientists are typically an integral part of the marketing and planning process to unravel useful insights and derive statistical data for planning, execution and keeping track of result-driven business strategies. Training a resource as a data scientist means having abilities to think and operate as scientists, delving deep into the art of rigorous hypothesis testing and experimental design best practices. Leading data scientists across the globe have the experience of dirtying their hands with real-world data taken from the industry to draw actionable insights about that data that can be effectively leveraged by the business for future.
Data Science is a complex field but not rocket science. It needs all the diligence, perseverance, is intellectually taxing and requires sophisticated integration of talent, tools and techniques. But a true data scientist is the one who can cut through these complexities and yet provide a clear, effective way for this business world to employ insights for value generation.
Having the aforementioned skills is what makes professional Data Scientists so sought after in any industry worldwide.
For years, CMO’s, CSO’s & various business leaders and IT organizations have had a perennial cold war going on. On one hand, IT half keeps struggling to keep up with round-the-clock technological shifts happening, inability to communicate true value to business, skill shortage, and many other unforeseen challenges. On the flipside, business leaders have to adhere to stringent project completion timelines, effectively manage budgetary constraints, and always keep looking up to the technology team yearning for continual support and to drive business more effectively. Data scientists, in many ways, act as a bridge between the two sides by extracting data from one & delivering relevant, contextualized insights to the other, which just understands the business language.
Data science today is often equated to software engineering primarily because it is code written. However, they are nowhere close to each other. Methodologies such as agile, waterfall, scrum etc are not easily embeddable methodologies that can be coupled with data science. Data science is more science and less engineering; therefore it should follow a more scientific method. Even umpteen times statisticians, data miners, data analysts are put at an equipotential with data scientists. Let me clarify here the basic underlying difference here on how Data Scientists operate differently. A modeler is typically has a defined scope & data they are supposed to be acquiring, analyzing and working with. Typical terminology associated with them is linear regression, logistic regression, Known distributions, confidence intervals, predictor variables and goodness of fit. On the contrary, Data Scientists are primarily driven by an essential tendency of human nature, our insatiable curiosity and the need to find answers to hardest of the problems. Data scientists are inquisitive, have a knack of asking questions which may be not so intuitive to the business at first go, do extensive “what if” analysis, question the existing underlying assumptions and business-as-usual processes. Armed with data and analytical results, a top-tier data scientist will then communicate informed conclusions and recommendations across an organization’s leadership structure. For them the world produces data in a black box (associated generally with algorithmic modelling) and often their vocabulary has machine learning, AI, neural networks, random forests, SVM, unknown multivariate distributions, iterative analysis, predictive accuracy etc. A data analyst may view data in silo from a defined source e.g. CRM, survey etc. A data scientist typically operates differently and mostly examines data from numerous disparate sources. They are expected to sniff all the incoming data with the intent of uncovering some hidden insight which may add tremendous value to the business. A data scientist looks at the data from a different eye, does much more beyond the usual reporting and contextualizes the insights in a form to business users which may see applicability. In a nutshell, they carry a strong business acumen, communicates well with IT & business side, simplify complex concepts into understandable information nuggets, know analytics & modeling in & out, are adept at handling data and can be called out as part analyst, part artist.
A typical day in a life of a Data Scientist involves performing historical data review and preparation (missing value estimation, outlier detection, descriptive statistics) followed by Data Segregation (training and validation set) and Variable Selection (checking multi-collinearity, selecting important variables). Post data massaging, the next key step is to build predictive algorithms (logistic regression/random forest/decision trees/K-means clustering/sequence mining/text analytics) and review results to refine the model (model diagnostics review). Model finalization is the last piece to the puzzle post which usual questions pertaining to propensity to buy, customer churn, channel optimization, customer lifetime value etc. can be answered.
Let me take a quick example of how a Data Scientist could add value beyond the visible boundaries & across the value chain of any business. Imagine an online retailer intending to build a recommendation engine that renders a whole new customer experience, promotes specific products based on current trends, browsing behavior, past purchase history and sentiment analysis. A typical solution is expected to increase both the conversion ratio and the average basket size. A data scientist in most cases would grow beyond his usual role firstly to explore data available in the public domain as well & may also deliver insights on the supply or the procurement side, how to avoid inventory stockouts, what are the right pricing strategies for a certain segment of customers, placement of certain SKU’s etc. He may come up with specifics around how the retailer can identify previously undiscovered products for cross-selling opportunities? Where can we find new revenue streams to offset the decline in revenues from certain channels? From a vendor management standpoint, it might be, who are the vendors to be reached out to service a given online order with minimal turnaround time and optimal costing (based on the order delivery committed to the customer in next 3-hr, 1-2 days, 3-4 days).
Data Science has already been creating an impact in every aspect of our lives, from preventive healthcare management, to rehashing internal business processes, to mitigating risks effectively, and even the convenience of having highly relevant, hyper-personalized experiences on ecommerce websites. Hopefully this article would have shared some perspective to how these extra-ordinary thinkers could truly impact businesses across the board for multiple industries by asking the right questions and gleaning into not-so-ordinary data from different realms of business. Waves of change have just begun. Data science has lot more to offer than what we could imagine sitting at this point of time & surely as we move forward in 2015, there would way more exciting applications of Data Science getting unraveled. Do share thoughts/comments/experiences on how Data Science added value or can add value to your business.