Data Science Maturity Quotient (DSMQ): How important is this for Enterprise Growth?
3AI April 7, 2021
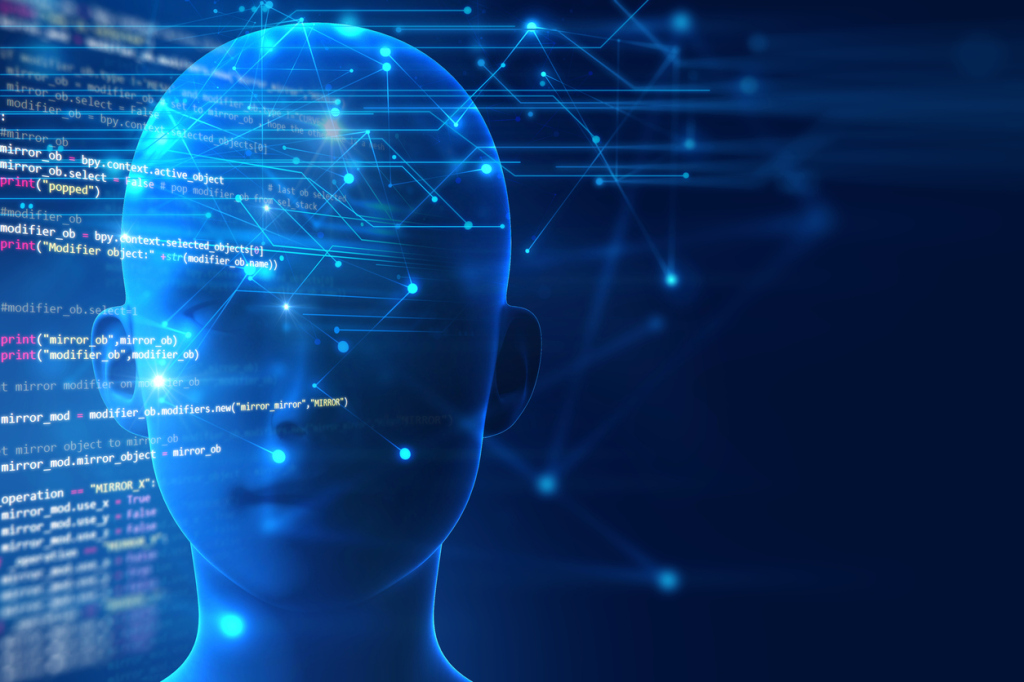
Most organization do not take full advantage of the power of their data. They use it in only basic ways, such as creating reports and analyzing past trends. But what if they could do much more? What if they could find hidden patterns and ocnnections across all of their data? What if they could use those insights to anticipate future trends? What if they could take it yet a step further, creating thousands of possible scenarios and then identifying the ones most likely to achieve the organization’s specific goals?
This is the data sciences maturity, the step-by-step process through which an organization gradually gains more knowledge about its data to create new opportunities and solve complex problems. It is comparable to how an organization traditionally gains insight: by continually testing and refining its institutional knowledge with new information and experience.
This data science maturity is measurable by what we defined as Data Science Maturity Quotient (DSMQ) with key dependency on data quality (DQ), data-driven leadership (L), people with analytics skills (P), data-driven decision-making process (D) and agile infrastructure (I)
(DQ) – DATA QUALITY
Data Science starts on solid foundation of good data. Organizations who have invested in infrastructure with appropriate data collection and storage process have laid the ground work needed to start leveraging data as an asset. Bad data quality can severely impair an organization’s ability to learn about their customers and their products through data. Hence data quality is the foundation on which analytics stands.
(P) – PEOPLE
Next comes, people with the right analytical skills, both technical skills to analyze data and interpersonal/business skills to bridge the gap from data to business. Investment in data science training (most analysts don’t have background with formal analytics training) and hiring senior data sciece professionals to lead the team moves the organization another 30% towards analytics maturity.
Collaborate With Organizational Change Management Teams to Design a Holistic Approach to Data Science Skills Development
Product training offered by vendors and system integrators at time of purchase focuses on the features and functions of the tools. Meanwhile, enterprises deploying analytics solutions spend much of their energy and resources on implementing these analytics tools and building analytical data models.
However, our client interactions suggest that organizations achieving analytics excellence spend as much or more time making necessary changes to improve staff effectiveness and adapt key processes. Organizations with lower analytics maturity are most successful with analytics when they build solid foundational competencies for data and analysis — such as understanding the context of the data they are using, the impact of data quality and the relation of key metrics with business decision making. Organizations that are more advanced with analytics are able to maximize the use of sophisticated analytics solutions, models and algorithms, and make effective use of data scientists.
Linking data and analytics to behavioral and cultural change is emerging as the next challenge to addressing the full potential of the digital workplace. Gartner identifies analytic culture as one of the four “pillars” of a comprehensive business analytics program.
The purpose of analytics is to enable evidence-based decision making, stimulating change actions that drive additional and measurable business value. In effect, a business change is implied whenever analytics are deployed. The impact on people and on behavior is potentially significant, and not always immediately obvious.
Organizational change management skills are therefore crucial for success. Moving to a higher level of analytic maturity involves initiatives that are enterprisewide and strategic, and that often span different geographic locations, time zones, cultures and business units. To achieve change toward a data-driven culture, developing a holistic and business-oriented approach to data science skills is integral.
(D) – DECISION MAKING PROCESS
Once leadership and people are in place, then data needs to be inserted into the decision making process. As an example, how a company allocates marketing budget is a good representation of whether data has a place in the decision making process. Is it zero-sum based? i.e. $’s allocated based on expected ROI. Or is it status quo per last year and some incremental. (I.e. data is irrelevant in decision making). Data-driven decision making makes up 20% of the DSMQ pie. Also, deciding on how to progress the decision making process as far as Capability is concerned is a curcial aspect.
Building the Data Science Capability Maturity
Data Science Capability Maturity is characterized by five stages, each stage a necessary building block for the next. The first two stages are Collect and Describe, in which organizations gather data and organize it in a useful way. Most organizations are at this stage, where data analysis tends to focus on what happened in the past.
The next stage is Discover. Organizations use the data they collected and described to search for valueable patterns and connections. This kind of knowledge can be game-changing – in detecting fraud, for example, or in discovering a business opportunity that competitors do not see.
The insights gained in the Discover stage prepare an organization for the next stage, Predict. Here, data scientists use computer models to create and think through any number of possible scenarios, and then determine which ones are most likely to occur.
In the final stage, Advise, data sciences are used to sift through the most likely scenarios and recommend the optimal course of action based on the organizations resources and goals. Ultimately decision makers will still rely on the collective wisdom of their organizations – that does not change. But now, they can tap the collective wisdom of the data as well.
( I ) – INFRASTRUCTURE
The remainder 10% comes from investing in agile infrastructure for ease of instrumentation i.e. as one does analytics and there is need for previously unavailable data, is there an agile infrastructure in place to quickly enable capturing of new data field and sources. The new data source or field then feeds into the foundation with which we started i.e. data quality.
So analytics maturity is a cycle that feeds into itself. Each incremental step enables higher degree of maturity in other dependent areas. The biggest hurdle organizations face, as they embark on the analytics journey, is data-driven leadership. Once that is in place, and they focus on bringing in right analytics skills, often rest falls in place.
(L) – Leadership
Once the foundation is laid, one of the first things needed is leadership that believes in leveraging data for making decision. Data-driven leaders not only trust data to prove/dis-prove their own beliefs about business opportunities but also are open to learn from data, of their beliefs. Once the organization has majority of decision makers wired to leverage data, 40% of the battle is won.
Identify And Communicate A Common Vision And Target State For Data Science
The overall end-state and business expectations for data science capability will need to be defined and socialized. It is a common and wrong assumption that everyone understands, and is on board with, the concept of data science and evidence-based decision making. Establishing the expected target state helps ensure that both the IT department and business leadership have a common vision of the transformation, and are able to articulate the rationale for the adoption of analytics and maintain focus of the data science program. Establishing the desired business outcomes for analytics is foundational to the learning process and ensures that any training is carried out within the context of the business plan. Any change plan will also incorporate sufficient flexibility and adaptability to enable the organization to respond to changes in market dynamics
Prepare for cultural-readiness education
Overall organizational readiness for change is important. A low level of readiness to be a data-driven/analytic business can be a major inhibitor. Evaluate analytics readiness using a maturity assessment such as Gartner’s ITScore for Business Intelligence , and then prepare for a program of information awareness, education and data literacy prior to delivering more in-depth analytics training.
Final Calculation of Data Science Maturity Quotient
Speaking in mathematical terms,
DSMQ = DQ x (0.4 x L + 0.3 x P + 0.2 x D + 0.1 x I)
DQ represents data quality with value between 0 and 10. Sufficiently accurate data is foundational to data science and poor data quality would handicap any organization wanting to leverage data for decision-making
L stands for the degree to which the leadership is data driven and has a value between 0 and10. 0 being organizations where there are no leaders who believe in leveraging data for decision making and 10 for organizations where all the leaders are data-driven
P is the degree to which organization has people with right analytics skills. The analytics professionals use structured process for delivering efficient analysis with actionable recommendation to drive business impact. This too, takes value between 0 and10
D represents the degree to which the organization has data inserted within decision making process and takes on values from 0 to10
I, represents the organization’s readiness to instrument quickly and takes on value from 0 to10.
You will get a score out of 100, which could give you a very precise estimate on your data science maturity.
At the end of the day, customers are central to our business. Organizations which leverage data science and evidence-based decision model to understand their customers’ needs and behavior, and transform their offering accordingly, will fast gain market share and popularity.