Application of Reinforcement Learning in Supply Chain
3AI April 26, 2022
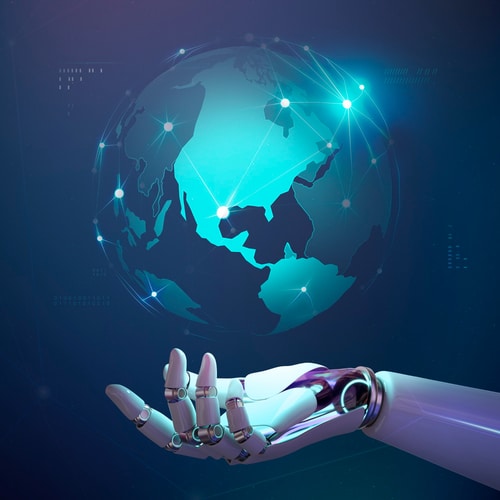
Author: Anindya Bera, Senior Manager – Anaytics, Genpact
A supply chain is a complex network of individuals and agents who exchange materials or information in a business ecosystem. The items which are used on day-to-day basis are created by a global collaboration between suppliers, manufacturers, and logistics carriers. What makes this complex? It is the network which contains several constraints, and the agents must constantly work against these constraints to maximise their gains.
Even moderately large businesses often handle 100,000 different products that could be spread over a large geographical area. Objective of such businesses would be to transport products to where they would be needed the most. Unfortunately, purchases can depend on various external factors beyond any businesses control – customer demand, availability, and global situation. This is applicable to almost all businesses even the ones that don’t operate in B2C space.
Challenge
Stable geo-political conditions together with improving infrastructure for the past decade have led companies to a false sense of security and they have expanded their supply chain to far reaches of the globe in the hunt for low cost. The events of the past two years have come as eye opener to all. Both the pandemic and war on the doorstep of Europe shows tails-events risks are real. Companies are now wary of risks involved with the globalized supply chain and impact of shocks is having on them.
In today’s world, modern products often incorporate critical components or sophisticated materials that require specialized technological skills to make. It is very difficult for a single firm to possess the breadth of capabilities necessary to produce everything by itself. The dependency on the global supply chain is more than ever for the producers as well as the consumers.
Supply-chain problem can be broken down in the following pieces. Demand variations – demand can surge due to planned promotion or due to any other unplanned factors. This can lead to situation of stock-outs that is there is not enough products in the inventory to meet the demand surge. Current microchip shortage is a prime example as result of which we are witnessing stock-out in industries like automobile. These situations can also be classified as demand shocks or a temporary sudden change in demand caused by any factors that:
- Allows consumers to consume more
- Induces consumers to want to consume more
The duration of the effects of demand shocks can vary greatly. Though there are no rigorous guidelines to define “temporary” , it is used to present the notion that the economy is in an irregular state and the aggregate demand is different from what economists consider as standard.
Wastage – this happens when there is an excess supply of a product compared to demand, especially if the product is perishable then it can lead to loses. Over-stocking leads to fight for inventory storage space. Companies tend to have multi-level of warehousing structure to prevent stock-outs and over-stocking, though might prevent stock-outs in theory it is not an efficient systems and lead to high operating cost.
The majority of supply chain automation efforts rely on heuristics or rule-based systems. Because the goal of each business is different, this approach necessitates the creation of hand-tuned heuristics for each instance. Furthermore, it is difficult to define heuristics for concurrently optimising end-to-end supply chain operations. As a result, most of these solutions tend to optimise in silos, without taking into account all of the constraints and agents in supply-chain networks.
Solution
When an organization goes through digital transformation and becomes data-driven, the reliability of its E2E pipelines and the quality of the data across all the pipelines become extremely important. This, in a
Reinforcement learning works best in problems that require multiple, sequential decisions to determine the optimum strategy or policy. Reinforcement learning models actively learn when agents move product or information across the network and arrive at an optimal stocking strategy or given system constraints. As a result, it is best suited to solving this problem. It provides a way to coordinate multiple agents – supplier, manufacturer, carrier, and warehouses – while maximising system gains.
Reinforcement learning outperforms the other methods because it makes the fewest assumptions while learning from data. As a result, reinforcement learning is better at adapting to dynamic environments on its own.
Because reinforcement learning is self-adapting i.e. the network adjusts on its own, it can handle the addition or removal of agents such as products or warehouses in the supply-chain better than other methods. The addition or removal of a warehouse, product, or supplier is a very typical phenomenon, but it is very difficult to account for the change in other solutions without human adjustment to the model output.
Modelling Approach
A simplistic way to model for this would be model each warehouse, suppliers, and stores as distinct agent. Each store (agent) then interacts with the warehouse to replenish stock based upon current inventory levels, forecasted sales, estimated restocking delays, and predicted wastage. The warehouse operates with similar inputs and outputs but interacts with suppliers. Rewards can be designed independently or jointly to maximize the desired goal, like a product availability or stock level.
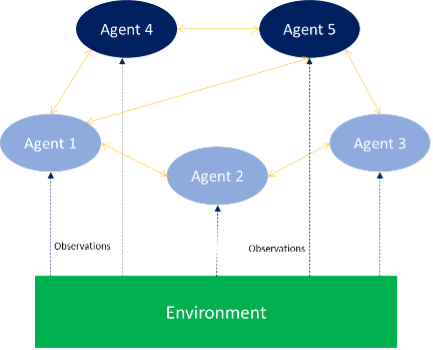
Since we are trying to model for a dynamic network where actions of one agent have ripple-effect all over the network. Model can then be extended by allowing the agents having complete knowledge of actions of other agents in the network. This allows agents to collaborate among themselves to leverage the learnings of other agents. Finally, agents not just have to optimize for local conditions like product availability but also have global constrains to maximize profit or minimize operating cost. The primary benefit of this approach is that it minimizes the “Forrester Effect” in the network, as system will be able to adapt to demand shocks which is pushed into the system by putting in a large order.
Conclusion
Reinforcement Learning may appear intimidating at first, yet it is a sub-discipline of Machine Learning. When compared to other supervised/unsupervised models, reinforcement learning models are challenging to implement; but, with prior ML competence and software engineering expertise, these solutions can be implemented. The availability of data and carefully stated goals, as with any other data science project, will be critical to success.
Reference
Reinforcement Learning for Multi-Product Multi-Node Inventory Management in Supply Chains – Nazneen N Sultana, Hardik Meisheri, Vinita Baniwal, Somjit Nath, Balaraman Ravindran, Harshad Khadilkar
How to Improve your Supply Chain with Deep Reinforcement Learning – Christian Hubbs
Inventory Control and Supply Chain Optimization With Reinforcement Learning – Phil Winder
Title image: freepik.com