A Greener Future with AI: Minimizing Carbon Footprint for Sustainable Innovation
3AI November 28, 2024
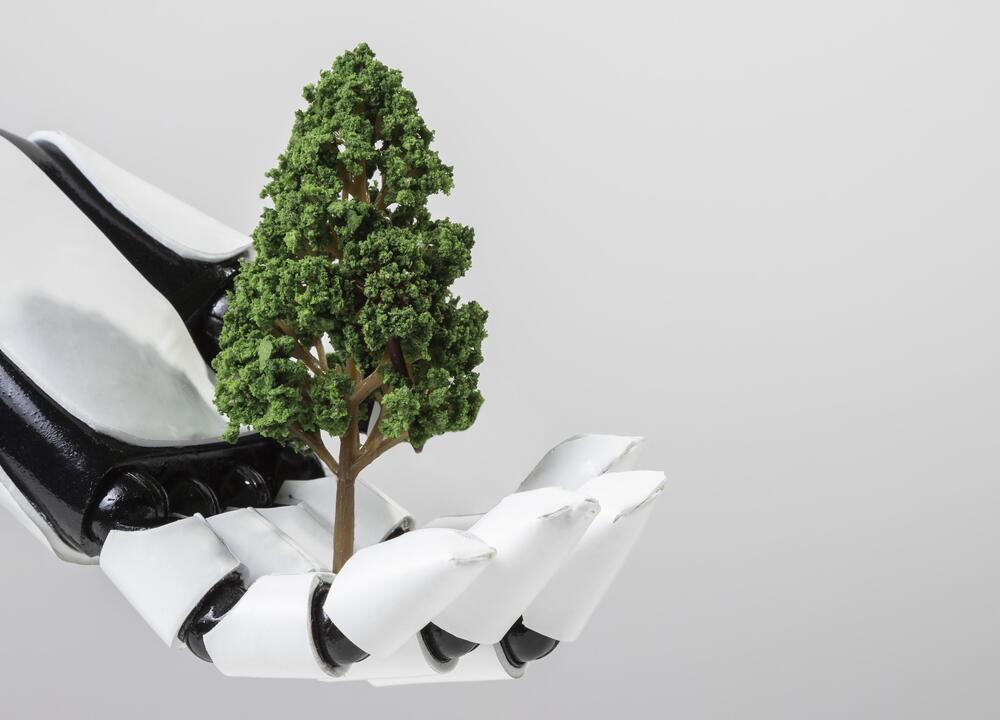
Featured Article
Author: Jemima Joy, ProV International
Artificial Intelligence (AI) has transformed industries and opened doors to remarkable advancements, from generative art to complex decision-making systems. However, AI’s rapid growth comes with an often-overlooked environmental cost—its carbon footprint. As the demand for AI-powered tools grows, so does the energy consumption required to develop, train, and deploy these systems. Understanding AI’s carbon footprint is crucial for creating a sustainable future where technological progress does not come at the expense of environmental well-being.
This article explores the factors contributing to AI’s carbon footprint, the specific environmental challenges posed by training large models, and the strategies being implemented to reduce these impacts. By prioritizing energy-efficient approaches, leveraging renewable energy, and encouraging transparency, AI developers and stakeholders can make more eco-conscious choices to mitigate the carbon costs of AI.
1. The Energy-Intensive Nature of AI Model Training
The development of complex AI models, especially large language models (LLMs) and deep learning architectures, requires substantial computational resources. These resources often reside in powerful data centres that house thousands of specialized processors, such as GPUs (Graphics Processing Units) and TPUs (Tensor Processing Units). These processors are critical for handling the vast quantities of data and complex calculations required to train AI models.
Training models like GPT-3, for instance, is not a small undertaking. The power demands for training GPT-3 were estimated to have produced hundreds of tons of CO₂. This carbon output is roughly equivalent to the lifetime emissions of five average American cars, highlighting just how energy-intensive AI training can be.
While GPT-3 is only one example, it is part of a larger trend: the training and fine-tuning of AI models often require multiple data centres running continuously for weeks, consuming massive amounts of electricity and contributing significantly to the industry’s overall carbon footprint.
2. Hardware Manufacturing and Maintenance: Hidden Carbon Costs
The environmental impact of AI is not limited to the energy used during model training. AI’s carbon footprint extends into the manufacturing, shipping, and maintenance of the hardware required to run these complex computations. High-performance hardware, such as GPUs and TPUs, require rare minerals and have an environmentally taxing manufacturing process. Additionally, these chips have a limited lifespan due to the heat and wear-and-tear caused by continuous, high-intensity computations.
Data centers housing this hardware need large-scale cooling systems to prevent overheating, which adds another layer of energy consumption. Cooling these systems uses a considerable amount of electricity and, in certain cases, water resources. When AI operations take place in water-scarce regions, this can create additional environmental and social pressures.
3. Reducing Carbon Footprint in AI Through Efficient Algorithm Design
One of the most effective ways to reduce the environmental impact of AI is through efficient algorithm design. The complexity of AI models contributes directly to energy consumption, so designing models that require less computational power without sacrificing performance can drastically reduce their carbon footprint. Researchers are experimenting with various techniques to make AI models leaner and more efficient:
- Model Pruning: This technique involves removing unnecessary parameters from a model without compromising accuracy, thus reducing the model’s size and the computational power needed for training.
- Quantization: Quantization involves using fewer bits for calculations, which reduces the energy demands of running the model.
- Knowledge Distillation: This process trains smaller models (or “student” models) to mimic the behavior of larger models (or “teacher” models), reducing the training load by creating a lighter version of a previously trained model.
Efficient design strategies like these allow for a lower carbon footprint while still delivering effective AI solutions.
4. The Rise of “Green AI”
As the environmental cost of AI has gained visibility, the concept of Green AI has emerged, aiming to reduce the carbon footprint of AI development by prioritizing energy efficiency. Green AI advocates encourage researchers and developers to consider not only the accuracy and performance of their models but also their environmental impact. One of the foundational principles of Green AI is transparency: by disclosing the energy consumption and carbon footprint associated with training AI models, researchers can raise awareness and allow consumers, companies, and other stakeholders to make more sustainable choices.
Some academic journals and conferences have started requesting energy consumption disclosures when submitting papers, which has fostered a culture of responsibility within the AI research community. This move toward transparency enables a standard of accountability and encourages researchers to explore less resource-intensive methods.
5. Renewable Energy: Powering Data Centers Sustainably
A promising avenue for reducing AI’s carbon footprint is powering data centres with renewable energy sources, such as wind and solar power. Many tech giants, including Google and Microsoft, have made significant commitments to carbon neutrality, pledging to offset their emissions by investing in renewable energy. Google, for instance, has already achieved carbon neutrality and aims to run its data centres on 100% renewable energy.
However, the transition to renewable energy faces logistical challenges. Solar and wind power are not always available consistently, which can make it difficult to meet the uninterrupted energy demands of large-scale data centres. Despite these challenges, ongoing advancements in energy storage and grid management offer hope for a future where AI-driven data centres can rely predominantly on sustainable energy sources.
6. Model Sharing and Lifecycle Management
Another impactful strategy for reducing the carbon cost of AI is model sharing and lifecycle management. Training a model from scratch consumes significant energy, so reusing and fine-tuning existing models (known as transfer learning) can save both time and energy. This approach is especially useful when deploying AI models for specific tasks, where a pretrained model can be adapted instead of creating a new one from the ground up.
Lifecycle management also includes decommissioning models that are no longer in use, as well as optimizing model storage to prevent unnecessary energy consumption. Researchers are also exploring ways to integrate carbon cost awareness into AI development, enabling users to weigh environmental costs when selecting models or approaches.
7. Ensuring Transparency and Accountability
As the industry works toward a more sustainable future, transparency and accountability are essential. By disclosing the carbon footprint of AI models, developers and companies can create a more informed AI ecosystem where environmental impact is as much a consideration as accuracy and performance. Standardized carbon reporting can also encourage competition among AI developers to produce the most environmentally efficient models.
Transparency also empowers consumers and stakeholders to make informed choices. By understanding the environmental cost of different models, companies and individuals can choose to support more sustainable AI solutions. It sets a precedent for responsible development and motivates further research into eco-friendly AI practices.
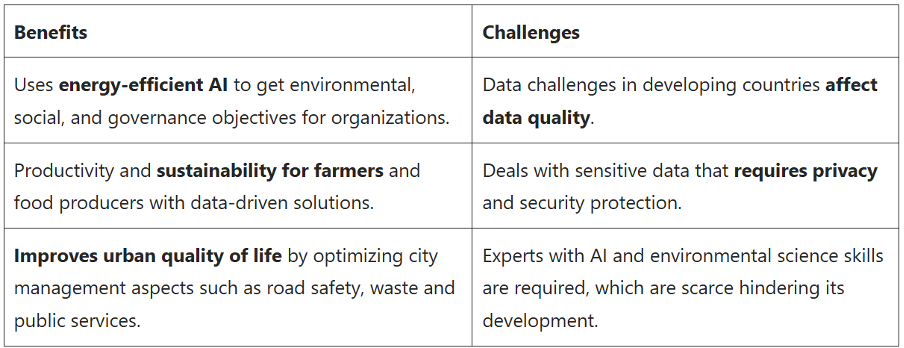
Looking Ahead: A Greener AI Future
AI’s potential to transform industries and improve lives is undeniable. However, as we continue to explore and expand the capabilities of AI, it is vital to address the associated environmental costs. By prioritizing energy efficiency, embracing renewable energy, and implementing transparency practices, the AI community can take meaningful steps toward a greener future.
Moving forward, the collaboration between researchers, companies, and policymakers will be critical to balancing innovation with sustainability. Efforts to fund research into energy-efficient algorithms, incentivize renewable-powered data centers, and encourage responsible AI practices all contribute to reducing the carbon footprint of this powerful technology.
AI has the potential to be a force for good not only in terms of technological advancement but also in promoting sustainable practices. With conscious, concerted efforts, AI developers can lead the way in making sure the benefits of AI are achieved with minimal environmental impact. By aligning AI’s future with the goals of sustainability, we ensure that AI-driven progress does not come at the cost of the planet we call home.
Title picture: freepik.com