ChatGPT and Large Language Models: A prioritization Framework for Leaders
3AI April 21, 2023
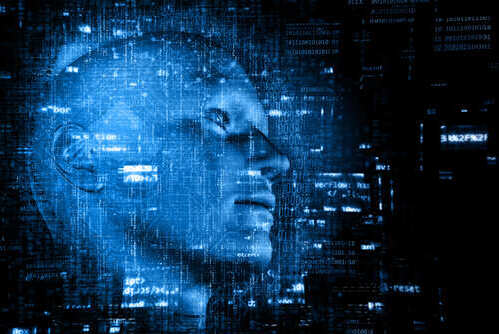
Featured Article:
Author: Robin John, Artificial Intelligence Strategist, APAC, Kimberly-Clark
Ever since ChatGPT was released last year, a flurry of LLM(Large Language Model) – related product announcements from tech giants have taken the world by storm. The key driving factor behind the success of ChatGPT is its human-like interface into AI. A technique called Reinforcement Learning with Human Feedback (RLHF) helped ChatGPT provide more “human-like” answers compared to earlier LLMs. Gradual improvements in intelligence combined with rapid improvements in conversationality enabled ChatGPT to cross a certain threshold of usability and the results are for all of us to see.
Being a conversational interface, ChatGPT is increasingly becoming a window for the world to access the powers of AI. Many people might be voluntarily interacting with an AI for the first time through ChatGPT. While it is beneficial to explore ChatGPT for a multitude of personal use-cases, it is important to have a curated approach while exploring potential enterprise use-cases.
In order to judge which use-cases would “click” for ChatGPT and LLMs in an enterprise, it is important to develop a framework to help analyze potential use-cases and also apply operational considerations before implementing them.
The framework
There are two key dimensions along which ChatGPT use-cases should be thought through. The first is the level of conversationality required for the use-case. Thanks to ChatGPT’s improved tuning to provide human-like answers, use-cases such as chatbots that require conversationality are hot favourites for implementation and rank higher on this axis.
The second dimension is the cost of error – i.e. how much would a wrong chat output cost an enterprise. For example, a bot providing medical advice should have a much lesser tolerance for factually incorrect responses compared to a ChatGPT-powered code generator. We should also take into account the ability to correct response errors by having a human in the loop before AI output reaches the end-consumer. For example, we have the opportunity to correct errors in a marketing copy generated by AI before publishing it but not in the case of a chatbot responding to a user query.
There is also an inverse relation between cost of error and the degree of creativity required. For example, there is very less creativity that should be possible in a medical advice generator whereas a content generation use-case could be all about creativity.
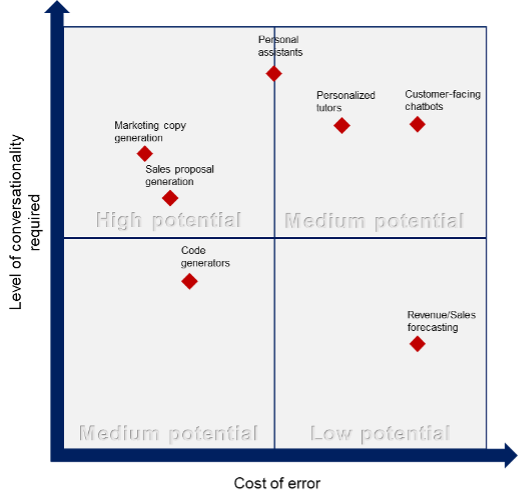
Figure 1 includes these 2 dimensions to create a framework which we could leverage to identify the best-fit ChatGPT use-cases for an enterprise.
It is also worth noting that a lot of traditional machine learning use-cases leveraging tabular data will likely fall into the low-potential quadrant. There might be just limited potential to make predictions and forecasts by making ChatGPT read tabular data. Traditional ML methods are likely to perform much better in these cases. Also, wherever conversationality required is less, it might be practical to explore some of the older LLMs that are likely to be simpler and more cost-efficient to implement.
Operational considerations
Once we prioritize ChatGPT-like capabilities, it is important for a decision-maker to be aware of certain key considerations that would impact delivery, especially if the path being pursued is to “build” and not “buy”.
- Training the model: Enterprise use-cases mostly would require “tweaking” ChatGPT to the enterprise context leveraging internal data. This could be achieved using prompt-engineering or embeddings. Each of these approaches have their unique advantages and disadvantages. Implementation teams should have conversations internally and with stakeholders before zeroing in on an approach. Practical implementations are likely to require some amount of “chaining” and “templating” to achieve right outcomes. Having a clear vision regarding training process would enable a faster path to production.
- Platform selection: Microsoft recently announced availability of ChatGPT as a service on Azure. There are other LLM platforms too but each of these LLM platforms are at different stages of maturity. Platform maturity, combined with their availability on an enterprise’s stack would decide how soon and how well can a use-case be implemented.
- Data privacy/Security : ChatGPT was a first of its kind and was clearly the tipping point in the LLM maturity timeline but there are other models too that could be looked at. BigScience’s BLOOM is worth looking at from a data privacy perspective. Databricks recently announced Dolly, with a “ChatGPT-like instruction following ability” but with a much simpler model that can be “directly owned” by enterprises. Enterprises should choose models basis their requirements around privacy and security. Aligning to a model that suits the enterprise priorities should help accelerate value delivery.
The current consensus is that the ChatGPT wave is comparable to the internet gold-rush of the late nineties. The ensuing bubble had triggered a lot of ideas and start-ups but soon there was a phase of consolidation as well. Similarly, it could be that a lot of potential ChatGPT ideas right now may not live up to the expectations. It is important for leaders to tread this wave with cautious optimism and spend investment dollars in use-cases with a clear path to value.
Disclaimer: Opinions expressed are solely my own and do not express the views or opinions of my employer