Mitigating Bias in AI Systems
3AI June 14, 2023
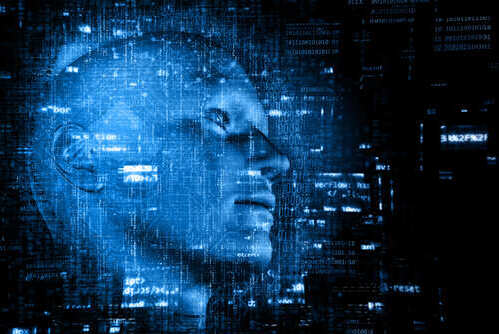
Featured Article:
Author: Kritika Saraswat & Sreedhar Sambamoorthi, AB InBev
Twenty Years ago, science fiction stories were ripe with futuristic scenarios with cars driving themselves, computer writing stories and human-like holograms responding to any and every query that we had. These scenarios were considered a figment of good writer’s imagination and books and movies on this opened to great reviews.
Cut to today, yesterday’s figment of imagination is today’s reality with AI writing stories and generating ART in minutes with such ease that it sometimes becomes impossible to distinguish on whether it was written by a human or AI. With products like Siri, Alexa and now ChatGPT, the chatbot universe is also taking the world by storm. AI today also has applications in Robotics, Defense, Transport, Healthcare, Marketing, Automotive Industry, Games etc. So the question beckons, can you imagine life without it? Absolutely not, and since with great power comes great responsibility, Responsible AI is the need of the hour!
AI is a Boon or Bane?
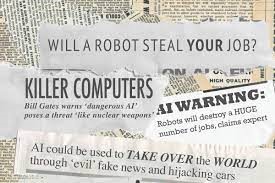
“The Development of full artificial intelligence could spell the end of the human race. […] It would take off on its own, and re-design itself at an ever-increasing rate. Humans, who are limited by slow biological evolution, couldn’t compete, and would be superseded.”
- Stephen Hawking
AI algorithms are capable of comprehending humans even more thoroughly than we comprehend ourselves. Every time when we watch something online or use google to search or publish something on social media apps, we are leaving behind some information. AI Algorithms can find patterns in the data and comprehend our motives, likes and dislikes.
Therefore, there are very high chances that AI can pick up society stereotypes and biases from real world data. There have been many instances where AI applications have made mistakes and faced criticism.
Here are some examples:
- A facial recognition software labeled two Black people as “gorillas”, later the label was removed from its software, but the incident highlighted the dangers of bias in AI systems.
- There have been reports of gender bias in a translator application because it was systematically translating gender-neutral pronounce to masculine pronouns in several languages, including English.
- In 2006, news article by ProPublica revealed that the compass tool, which is used in the United States to help judges decide whether to release defendants on bail, was biased against black defendants. The Tool uses an algorithm that predicts the likelihood of a defendant reoffending or skipping bail. The investigation found that the tool was twice as likely to incorrectly flag black defendants as higher risk, compares to white defendants who were wrongly classified as low risk.
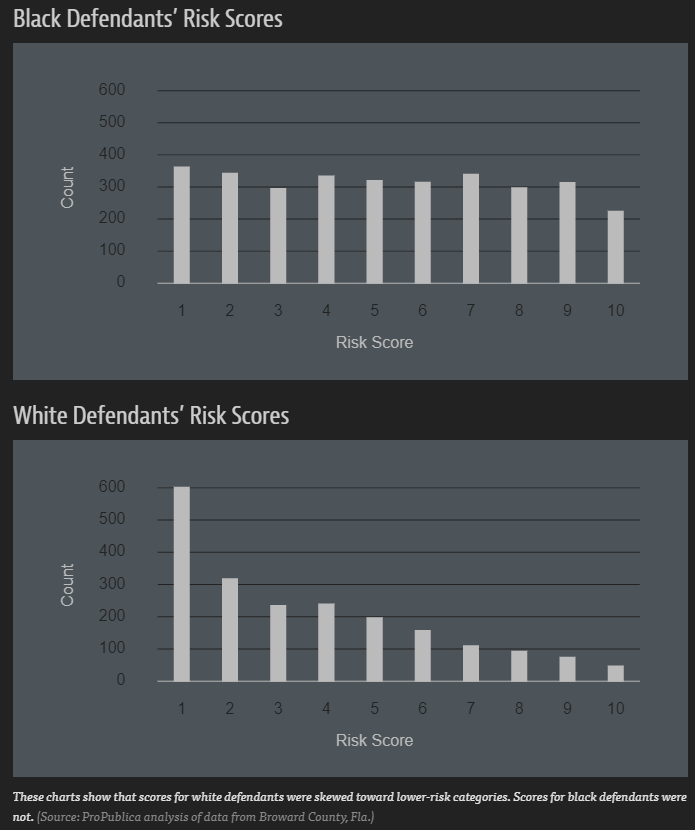
It is very clear that Artificial intelligence bias can create problems ranging from bad business decisions to injustice and this has sent organizations scrambling to provide guidelines for responsible usage of AI, and the term used for it is known as Responsible AI.
Umbrella of Responsible AI
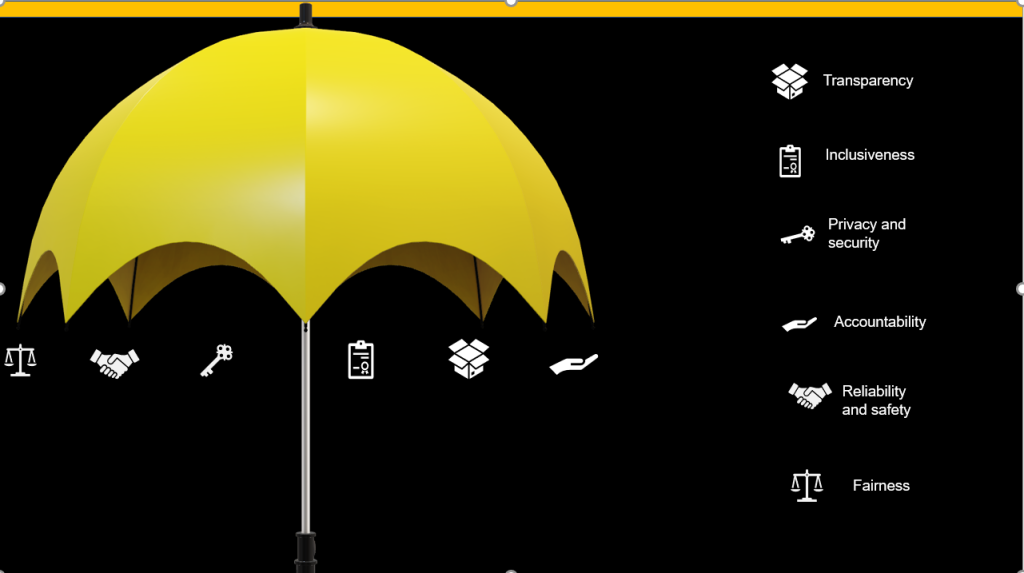
Some people see Artificial Intelligence as a sign of growth, productivity and some people see it as threat. Lack of unbiased decision, lack of privacy and security is a major concern and there is an urgent need for suitable guidelines and that is when Responsible AI comes into picture.
Responsible AI is an umbrella term for many aspects of making the right business and ethical choices when adopting AI.
It covers mainly 6 areas: Transparency, Inclusivity, privacy and security, reliability & safety, fairness.
Let us briefly look at some important terms
Fairness – Definition of Bias & fairness varies in different context, Lawyers and philosophers have different perspective of it, but in AI we can say that fairness is when AI system treats everyone fairly and avoid affecting similarly situated groups of people in different ways.
Reliability & safety – AI system should operate reliably, safely & consistently under normal & unexpected circumstances.
Privacy & security – AI system should be able to protect private information & resist attacks.
Inclusiveness – AI system should empower everyone and engage people.
Accountability – AI system should be responsible for the actions and developers should have Meaningful control over the AI system, and accountable to its action on the environment.
Transparency and Explainability– People should be able to understand how the decision has been made, it’s the concept that a machine learning model and its output can be explained in a way that “makes sense” to a human being at an acceptable level.
Methods of Interpretability:
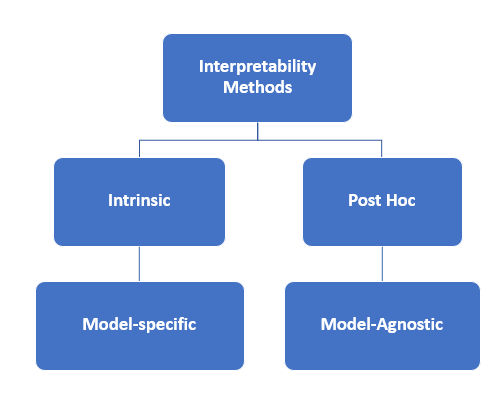
- Intrinsic – Interpretability is achieved by Restricting the complexity of the model.
- Model – specific – “Model Specific” tools only work for a specific model.
- Model Agnostic – Model-agnostic Tools can be used on any machine learning model. These methods cannot have access to model internals such as weights or structural information.
- Post Hoc – Interpretation of model takes place after model training.
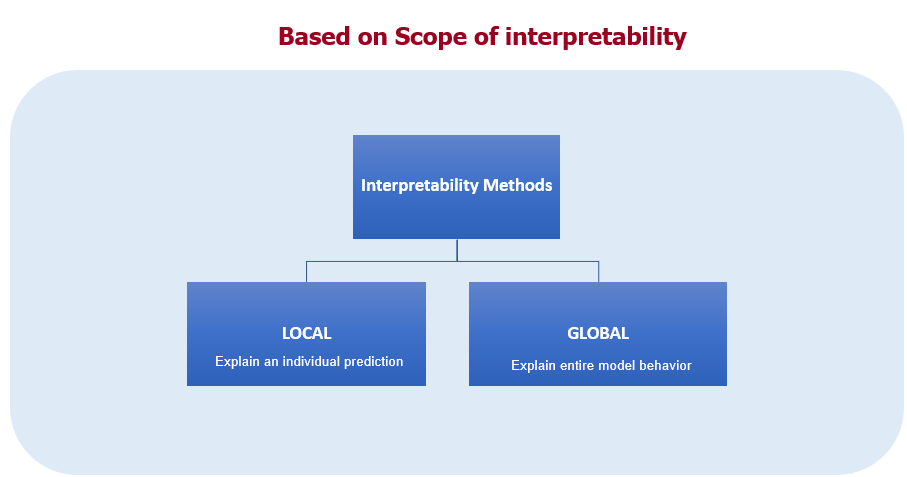
Where do we look for bias in ML application lifecycle & how do we mitigate it?
Bias can be found in almost every step in ML pipeline starting from task definition to deployment.
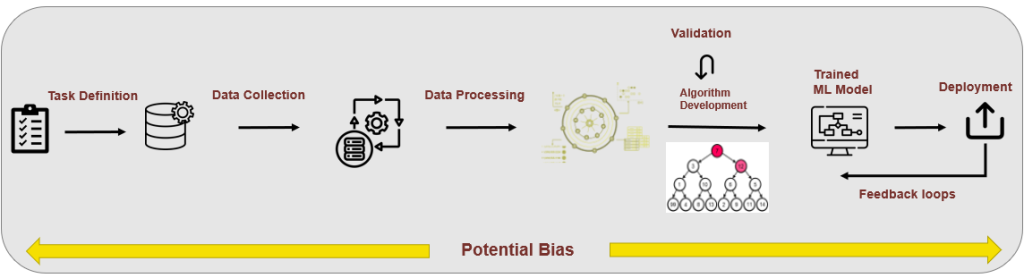
Task definition – Task definition should be defined clearly otherwise it can introduce bias. Example – The task of “predicting criminals based on face images” is highly problematic and can potentially cause harm to misclassifying individuals. Another example can be “Ranking job applicants based on their demographic attributes,” as it can perpetuate bias and discrimination in hiring processes. To mitigate it we should involve diverse stakeholders with multiple perspective.
Data Collection and preprocessing – Data is very prone to societal bias & skewed samples. we need to critically think about the subpopulation that we are taking, handle any technical, human bias or Imbalanced data and do hypothesis testing for different scenarios.
Bias in model – It is critical to select the right model for the use-case and make sure if model structure is not introducing bias. Define model assumption, be careful about Bias variance tradeoff. Explainablity tools like Lime, Shap, AIX360 can give us insights about model and data.
Deployment – it is crucial to identify AI bias before you scale. Check for datadrift which is variation in the production data from the data that was used to test and validate the model. Tools like – DeepChecks, Great Expectations or Evidently AI can help us with that.
In conclusion, it is imperative to acknowledge that while artificial intelligence (AI) holds significant promise for societal advancement, it is accompanied by ethical considerations. By embracing the principles of responsible AI, we can guarantee its conscientious development and responsible deployment, thus safeguarding its ethical integrity.
Disclaimer – This is the personal view of the author based on the experience while working with AB INBEV GCC Services India Private Limited (“GCC or ABI”) and hereby shared for your reference only. No Warranties, Promises and/or representation of any kind, expressed or implied, are given as to the nature, standard, accuracy or otherwise of the information provided in this article nor to the suitability or otherwise of the information to your particular circumstances.
GCC or ABI have no control on the contents of this article and shall not be liable for any loss or damage of whatever nature (direct, indirect, consequential or other) whether arising in contract, tort or otherwise, which may arise as a result from your use of the information in this article and will not accept responsibility for the accuracy of this article.
References –
https://learn.microsoft.com/en-us/azure/machine-learning/concept-responsible-ai?view=azureml-api-2
https://www.microsoft.com/en-us/ai/responsible-ai?activetab=pivot1%3aprimaryr6
https://www.propublica.org/article/machine-bias-risk-assessments-in-criminal-sentencing
Title picture: freepik.com