POV: Executive’s guide to build GenAI COE Effectively
3AI August 7, 2024
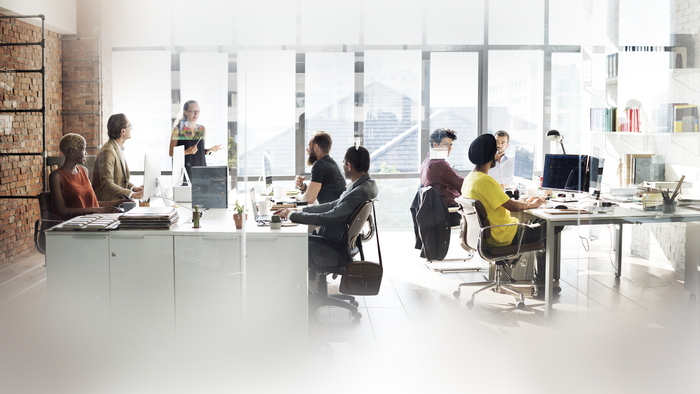
Featured Article
Author: Mausam Deb (Healthcare & Life Science – AI Leadership Consultant)
Generative AI (aka LLM) is no less than a rage for the last few years. The democratization of technology achieved by OpenAI through its GPT-3.5 model is remarkable. Market size of GenAI is growing in leaps and bounds. Every company in the marketplace, be it from core industries like Banking, Manufacturing, Healthcare, Life Science etc. or from System Integration, is trying hard to master LLMs and generate ROI. One of the tried and tested approaches of creating expertise in organization is through focused Centre of Excellences (CoEs). However, feedback from numerous business leaders within the B2B space indicates that the outcomes or effectiveness of GenAI CoEs have fallen short of expectations. A lot of that is a direct result of extreme marketing and oversimplification of GenAI.
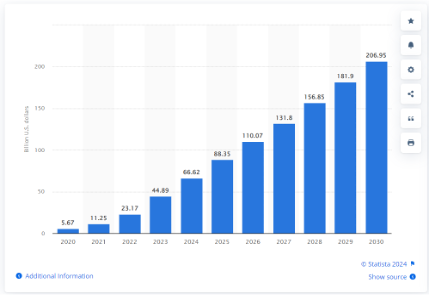
As professionals, we should be focused on both the possibilities and practicalities of GenAI implementations. So, let’s try to answer – How can we build GenAI CoE effectively?
Executive Framework of building GenAI CoE Effectively
1. Understand the Purpose and Scope of COE
It’s absolutely crucial to define the purpose and scope of the GenAI CoE. Many executives started / allocated funds to start GenAI CoE out of pure FOMO (fear of missing out). Unfortunately this sets the course towards frustration. Unfortunately, I have seen many executives are confused between doing a project or developing a POC using LLMs and building a Centre of Excellence for GenAI. These two are different problem statements. Whereas earlier one can be done using jugaad for one off cases but the later needs planning and establishing ground rules of repeatability, reliability, availability, acceptability and reproducibility.
If any company is either developing an inhouse GenAI solution or providing GenAI powered services, it’s absolutely important to understand the purpose, scope and alignment with the overall business of it. Because that will set the expectations early on
2. Be Patient to Develop the Right Skills
Most of cases, traditional Artificial Intelligence (AI) or Advanced Analytics Centers of Excellence (CoEs) have emerged as a result of the efforts of Data Engineering teams. While most organizations already had personnel with a basic understanding of these concepts. But we are still in the early stages of the Generative AI (GenAI) boom. Despite claims from marketing professionals that developing prompts is simple, which holds true in many instances, it differs significantly from the conventional method of interacting with data. In most cases, achieving accurate results is more challenging than one might anticipate.
“The initial step is to acknowledge that Prompt Engineering is a skill set that requires internal development, as it is not readily available in the current market.“
Numerous companies have asserted that they have trained a certain percentage of their workforce in Generative AI (GenAI) and are ready to make a global impact. However, merely deploying a Copilot through GitHub or via MS365 will not adequately train employees in GenAI. Before developing an effective use case, it is crucial to comprehend the concepts such as zero shot, n-shot prompting, chain-of-thoughts, RAG, embeddings, and very new context search and IR techniques. Additionally, technologies for utilizing prompt templates (e.g., langchain, langflow) and Vector Databases (e.g., elasticdb, chroma etc.) are relevant. A significant portion of the process, at least to date, involves trial and error.
Therefore, Patience is essential
3. Select the right POCs or Use Cases to justify ROI
A significant challenge with GenAI lies in its ease of initiation, contrasted by its extreme difficulty in scaling as a practical solution, particularly when high accuracy is required. Furthermore, its associated costs escalate rapidly, potentially exceeding initial projections. For a mid-sized organization, both the factors constitute substantial obstacles, diminishing the potential ROI.
Therefore, it is crucial to select the right use case with a high tolerance level for inaccuracies. Example.. embarking directly to revolutionize search functionalities may lead to unfavorable outcomes, unless the organization has exceptionally patient leadership.
“Selection of appropriate use cases for proof of concept is of paramount importance. I myself made this mistake. Initially GenAI evoked feelings in me akin to that of a child in a candy store, but as we went deeper it became evident that it was difficult to scale and hence ROI toppled“
Answering just few questions will lead towards selection of the right use case
- Is it something adding towards customer experience, cost reduction or new business line?
- Is it a marginal improvement for my product or adding a completely new dimension?
- Can we live with 40% accuracy and very less reproducibility?
- What-if the use case can not be scaled?
4. Select the RIGHT Partners to Scale
LLMs (GenAI) have surely taken the world by storm, but it is still far from being democratized. A handful of major powerhouses control LLM models, and it makes little sense for a mid-size CoE or Innovation Centers to fine-tune parameters because of sheer cost.
Even though there are only a handful of powerhouses, models (tex-to-text, text-to-code, voice, picture, multi modal etc.) are still fragmented. There are a growing number of features coming to the market that address this issue, e.g. some platforms allow users to select multiple models at runtime (e.g. AWS Bedrock), but the options are still limited. Open-source models require a significant investment of time and money, and it only makes sense if anyone is planning for the long term and ok to spend $2M+ to $4M+ for tuning just the model.
All of these factors make it expensive to train people in GenAI as well. Therefore, it is important to choose model, cloud, and service partner wisely. Look for a partner that can invest in training and support the business case, not just tell fascinating stories out of POC.
5. Develop Governance, Guardrails and Policies early on to drive Adoption
As we discussed in Point-1, developing a Centre of Excellence is significantly different from doing a POC or a project. COE needs to set-up the rules of engagement early-on to establish itself as the go to place for organization. Interestingly, GenAI changed the rules of the game completely.
….. COE needs planning and establishing ground rules of repeatability, reliability, availability, acceptability and reproducibility.
GenAI stands out from traditional AI in a significant way. In traditional AI, models are constructed, trained, and executed using the organization’s exclusive data. Conversely, LLM models leverage data from diverse sources (often referred to as collective data), while training is conducted on the organization’s own data and run on a shared computing ecosystem that is only promised to be secure.These factors make it important to select model governance that align with the organization’s specific use cases.
Due to the inherent characteristics of Large Language Models (LLMs), it is exceptionally challenging to mitigate security vulnerabilities and attacks effectively, necessitating the implementation of robust safeguards. While the field of LLM guardrails is an active area of research, unfortunately there only are limited comprehensive solutions available in the market. But this can be achieved through rule-based frameworks, LangChain Guardrail Library or frameworks like MS Spotlighting.
The policy landscape of LLM regulations is in its nascent stage and is evolving in a fragmented manner across different countries. Unfortunately, at present, there are only a few comprehensive compliance guidelines available, with the exception of a few European countries. Therefore, it is imperative to vigilantly monitor the regulations and compliance requirements applicable to the countries in which your products will operate.
Conclusion
GenAI and LLMs are active fields of study. All its guiding principles are evolving at a rapid pace, sometimes difficult to grasp. The above framework provides a directional guide that helped me in setting-up GenAI CoE but it is advised to do necessary tweaks depending on business / situational need.
Title picture: freepik.com