The Dynamic Duo of AI and Data: Unlocking Intelligent Solutions and Powering Future Capabilities
3AI July 28, 2023
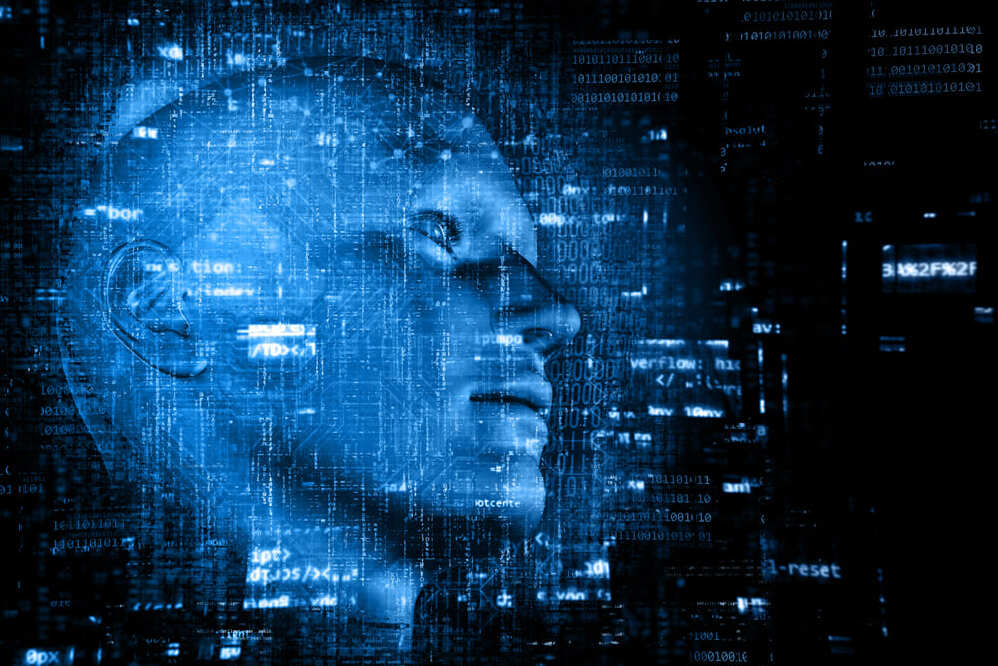
Featured Article:
Author: Manoj Suryadevara, Walmart Global Tech
Introduction
Artificial Intelligence (AI) and data convergence have emerged as a mighty force driving innovation and reshaping industries worldwide in this digital age. AI enables machines to simulate human-like intelligence while data fuel AI algorithms. This symbiotic relationship between AI and data is at the heart of the technological revolution, unlocking new frontiers in research, business, healthcare, retail, and multiple industries. This article aims to delve deeper into this dynamic relationship, exploring how AI harnesses the potential of data and how data, in turn, empowers AI to generate intelligent insights, accompanied by real-world examples and references showcasing their profound impact.
Data: The Crucial Fuel for AI’s Growth
The digital age has ushered in an unprecedented era of data generation, with 59 zettabytes of data created, captured, and replicated globally in 2020 alone. This data deluge serves as the lifeblood of AI, acting as the raw material from which AI algorithms learn and improve their performance.
Data is pivotal in supervised learning, a widely used AI technique. AI models use labeled data. Exposing the AI model to vast amounts of labeled data, it learns to recognize patterns and make accurate predictions. For example, training an AI model with millions of labeled images enables it to identify objects, animals, or even human emotions accurately.
AI: Unleashing the Potential of Data
Data, on its own, can be overwhelming and challenging to make sense of meaningfully. AI’s real strength lies in its ability to rapidly process and interpret vast volumes of data, turning raw information into valuable insights.
One prominent application of AI’s data-processing capabilities is Natural Language Processing (NLP). AI-powered language models can comprehend vast amounts of textual data, enabling sentiment analysis, topic modeling, and even understanding the context in which a utilization word or phrase occurs. NLP applications have revolutionized customer service, legal analysis, and healthcare diagnostics industries.
In the field of genomics, AI-driven data analysis has accelerated gene sequencing and gene expression analysis, leading to breakthroughs in personalized medicine and cancer treatment. By sifting through vast genomic datasets, AI algorithms can identify disease markers, predict treatment responses, and recommend tailored therapies.
Data Quality: The Backbone of AI Performance
While data is abundant, its quality is paramount. The saying “Garbage in, garbage out” (GIGO) underscores the significance of data quality in AI applications. Flawed or irrelevant data can lead to skewed insights and poor decision-making.
Consider a self-driving car relying on sensor data to navigate busy streets. If the data from these sensors is noisy or inaccurate, it could lead to potentially dangerous situations, jeopardizing the safety of passengers and pedestrians alike. Organizations need robust data governance strategies to ensure data quality, including data cleansing, validation, and verification processes. High-quality data is essential for AI models to deliver accurate and reliable results.
AI-Driven Data Analysis: A Game Changer for Businesses
The integration of AI with data analysis has revolutionized the way businesses operate. One of the most impactful applications is personalized marketing. AI-driven recommendation engines, like those used by Amazon or Netflix, analyze vast customer behavior and preferences datasets to suggest tailored products and content, enhancing customer experience and increasing sales.
Moreover, AI-driven predictive analytics has transformed supply chain management. Retail giants now use AI to forecast demand, optimize inventory levels, and streamline logistics, reducing costs and increasing efficiency. In finance, AI-powered algorithms can analyze vast financial datasets to detect fraud, assess risk, and optimize investment portfolios, empowering financial institutions with valuable insights for informed decision-making.
Ethical Considerations in AI and Data Integration
As AI and data become more pervasive in various aspects of life, ethical concerns surrounding their integration have grown. Issues like bias in AI algorithms, data breaches, and improper data handling raise questions about privacy, fairness, and accountability. AI models trained on biased data can perpetuate and amplify existing prejudices. For example, facial recognition systems trained predominantly on data from certain demographics may fail to accurately recognize faces from underrepresented groups.
For responsible AI deployment, it is crucial to address these ethical considerations. Transparent AI models, diverse and representative datasets, and continuous monitoring for bias are essential steps toward building fair and ethical AI systems.
The symbiotic relationship between AI and data is a driving force behind the ongoing technological revolution. Data provides the raw material that fuels AI algorithms, enabling machines to learn and make informed decisions. In return, AI empowers data with intelligent insights, revolutionizing industries, shaping businesses, and enhancing human lives. As we move forward, striking a balance between AI-driven innovation and ethical considerations will be key to leveraging the full potential of this powerful alliance for the betterment of society.
References:[Text Wrapping Break]
- LeCun, Y., Bengio, Y., & Hinton, G. (2015). Deep learning. Nature, 521(7553), 436-444.
- Provost, F., & Fawcett, T. (2013). Data science and its relationship to big data and data-driven decision making. Big Data, 1(1), 51-59.
- Goodfellow, I., Bengio, Y., & Courville, A. (2016). Deep learning. MIT Press.
- Mitchell, T. M. (1997). Machine learning. McGraw Hill.
- Davenport, T. H., & Patil, D. J. (2012). Data scientist: The sexiest job of the 21st century. Harvard Business Review, 90(10), 70-76.
- Zhang, C., Bengio, S., Hardt, M., Recht, B., & Vinyals, O. (2018). Understanding deep learning requires rethinking generalization. arXiv preprint arXiv:1611.03530.
- Chen, T., & Guestrin, C. (2016). Xgboost: A scalable tree-boosting system. In Proceedings of the 22nd ACM SIGKDD International Conference on Knowledge Discovery and Data Mining (pp. 785-794).
Title picture: freepik.com