Better portfolio analysis for PE/VC firms underpinned by Analytics
3AI November 27, 2020
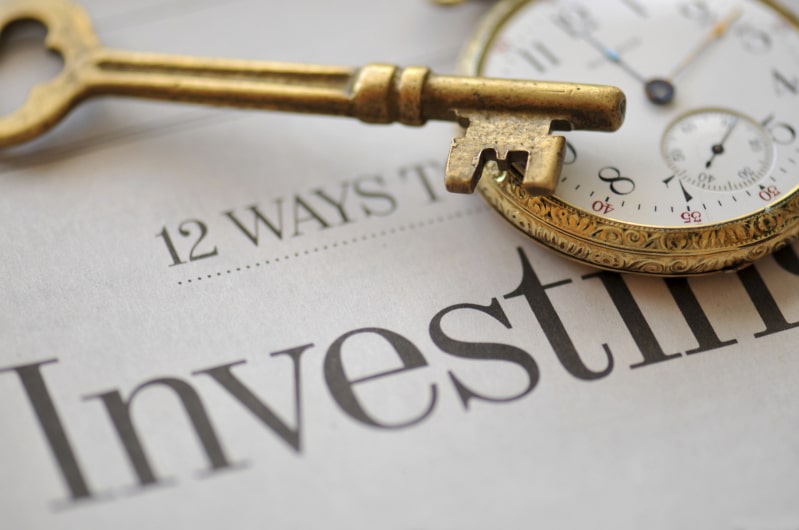
In the venture capital world, it’s all about the “hits.” A hit is a startup that makes it big, returning many multiples of a venture fund’s initial investment. Hits are great for everyone—investors, entrepreneurs, job seekers—but the problem is they don’t happen very often. The odds of a big hit are about one in 10.
Boosting the odds for VCs
But what if venture capital could boost its odds to 50-50, or even two out of three? With $48 billion in VC investment in 2014, such an improvement would prevent huge amounts of money from being lost on startups that never had much of a chance of surviving the harsh competitive environment. The challenge is to identify those likely laggards well before the market rejects their idea and, perhaps more importantly, to see the big hits before anyone else. Venture capital has long relied on subjective, intuitive methods of assessing startups, but that’s changing as more firms are bringing data science and consistency into their decision-making.
The next is to try to do the science, build the tools, and do the research all around this one question: How can we better predict when innovations will survive or fail, both for startups and when corporations launch new products or do acquisitions? There’s no human subjectivity involved anywhere along the line. All the algorithms converge on a discrete yes or no. That yes or no depends on majorly two areas – those inside the startup, and those external to the startup. Only around 20% of the predictive value to come from details specific to the startup itself whereas 80% comes from things outside of the startup, such as the market, customers, competitors, technology trends, and timing.
In this scenario, managers are trying to predict the future before investing money in it. According to analysis of data from the Small Business Administration, and data on startups, between 20% and 30% of new businesses survive to their 10th birthday. Startups with VC-backing, aren’t doing much better than spin-offs from large corporations. Has the entrepreneurship economy gotten any better at predicting any business? Data suggest no, not in any statistically significant amount. But Investment Analytics is turning that around. When it comes to predicting survivorship of companies after a 10-year period, implementing investment analytics at present setup can point you to the right answer 67% of the time, and totally wrong on the remaining third. So, If investment analytics has vastly improved the odds of investing in new technologies and businesses, why isn’t the entire VC world knocking on its door? Because A lot of venture capitalists, like the scouts in Moneyball reacting to sabermetrics, are skeptical. Most of the people don’t see quants taking over VC, even in the distant future, but they do see the potential of using data to help venture capitalists make decisions.
Data analytics are undoubtedly creeping into venture capital—Google Ventures uses an algorithm to help with investment decisions, and a Silicon Valley firm called Correlation Ventures is built upon an algorithmic investing strategy. But the old-fashioned process of detailed research and human judgment still has a lot going for it. Just ask the people at Lux Research, an emerging-technology consulting firm in Boston. For the past 10 years, Lux’s science-trained analysts have been scouring the business landscape for new technology firms, interviewing employees of those firms, and slowly compiling their own database of companies that succeeded or failed. Lux rates each company it profiles according to nine key factors, which are available to the public on its website in a report called “Measuring and Quantifying Success in Innovation. The result of that rating is a company profile with a “Lux Take,” which ranges from “strong positive” to “strong caution.”
The company recently looked back at five years’ worth of profiles and found that 50% of the companies that earned a “positive” rating went on to be successful, an outcome which Lux defines as an IPO, acquisition, or transition to standalone profitability. Given the usual odds of new business survival, the Lux system seems to inject a significant amount certainty into the process of evaluating startups. The company’s high rate of accuracy is attributable to two things: capability and methodology.
People talk a lot about the importance of innovation to economic growth. In a recent survey of voters in swing states by the Economic Innovation Group, 75% of those surveyed agreed that America needs more entrepreneurs and investors in order to improve long-standing economic problems. The innovation economy has an information problem. The information that drives it isn’t good. How can countries become innovation economies in a more efficient way? Let’s get better at funding the startup companies that will grow and drive employment. For every dollar that goes in the wrong place, that’s a bad dollar.
Better Investment Strategy for PEs
Data, and specifically the analysis of data is becoming a critical component in enabling private equity firms to create and maintain value for their investors. I know some of you are thinking, “What does a data management geek know about private equity?” One thing to note about private equity firms is that there has been a greater focus on IT and a growing need for data analytics services recently, especially for deals that involve middle-market companies and/or are add-ons to existing platforms. Increasing acquisition valuations and the dearth of available debt financing are making it increasingly difficult for private equity firms to generate outsized returns for their investors. This is causing private equity firms to focus more on operational improvements within their portfolio companies as a means of driving growth and value creation. Given the strong correlation between operational improvement and higher returns for limited partners, private equity managers’ hands-on involvement is, and will continue to be, critical to the future success of the fund. For private equity managers, this means identifying key drivers of EBITDA, understanding customer behavior, developing competitor analyses, determining the best place to invest capital, managing budgets, and controlling costs—for each of their portfolio companies. And to do it effectively and efficiently, managers must leverage data.
Nobody said it was going to be easy and the fact is: it’s not. Unfortunately, most of the private equity managers are not in a position to dig deep into the recesses of their portfolio company systems and extract the data tables necessary to produce key operational metrics. Therefore, managers will have to rely on the current workforce at each of their portfolio companies or hire an outside party to do the ‘dive into the data’ for them. Many of them struggle with capturing, extracting, and ultimately analyzing operational data within their businesses. This is largely due to the fact that, historically, middle-market companies have rarely made technology a priority, leaving them with an unsophisticated IT platform. As a result, when dealing with legacy and perhaps “home-grown” solutions, there are a variety of challenges that arise when attempting to harmonize, integrate, and perform data analytics. Additionally, many middle-market companies are experiencing data volumes that are growing exponentially and are held in disparate systems throughout the organization. In short, private equity managers are faced with massive amounts of “dirty” data that must be converted into meaningful metrics. This leaves private equity firm managers asking themselves, “How much time and money do I need to commit to information technology and data analytics?” Answer: more than you have in the past. The key is to invest wisely and in a way that will get you the greatest returns in the shortest amount of time. There are an infinite number of technology solutions out there. From off-the-shelf, in-the-box solutions to massive enterprise resource planning (ERP) solutions, software companies have done their best to provide an answer to your “big data” problems. Although there are many great tools out there, there is no cookie-cutter solution when trying to capitalize on the presumed synergies of integration. Private equity managers must understand the specific business needs and work to develop strategic analytical solutions driven from the data that already exists in their organization. It is critical, especially in today’s competitive market, to “leverage your data” and apply these targeting solutions in a way that will provide the greatest returns for your investors.
Big data may indeed be able to help, but it’s more likely to be a piece of the puzzle, not the solution. For instance, academic studies have shown that serial entrepreneurs successful in the past are more likely to do well in new ventures. That implies there is some explanatory power in looking backwards for guidance on what’s ahead. “But the nature of entrepreneurship is always changing,” says Josh Lerner, the Jacob H. Schiff Professor of Investment Banking at Harvard Business School. Most regressions predicting entrepreneurial success in the literature have very low goodness of fit, which suggests the limits of a ‘Moneyball’ approach here. Predicting which startup is going to be successful is much harder than [predicting] which baseball player is. It is as if the baseball rules are being changed every year in unpredictable ways.”