Data Science/Machine Learning and Software Quality
3AI March 29, 2023
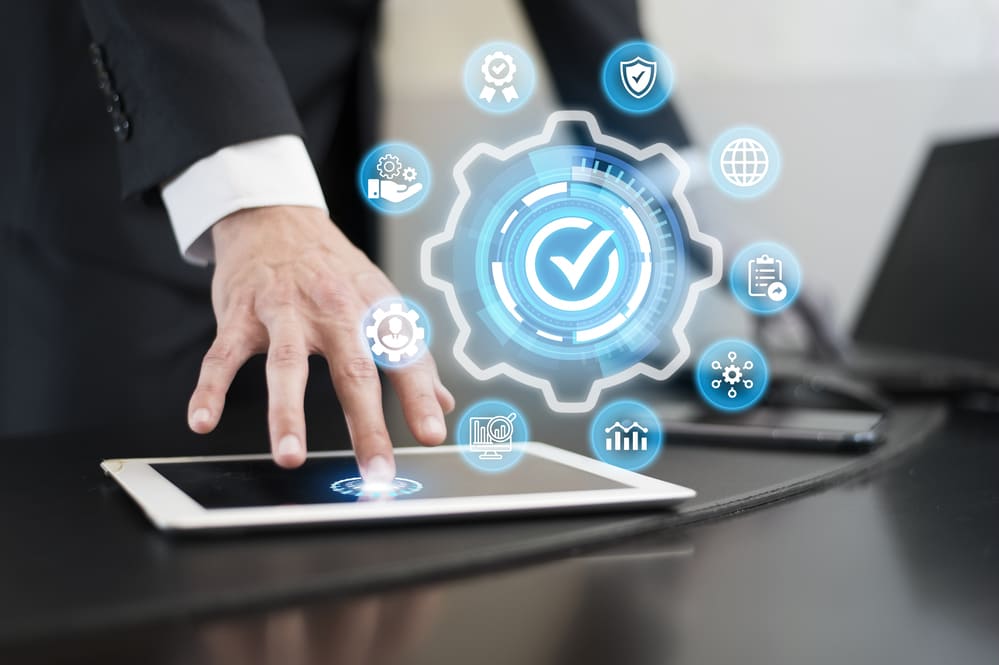
Featured Article:
Author: Biju Kalleppilli, Director-Performance Engineering, SAP Labs India
Endless possibilities of data science applications in Software quality are probably an underrated one.
At various stages of product testing life cycle, huge amount of data is generated and remain unexplored., primarily because the top management often become happy by looking at the quality metrics and test results. Let’s dig little bit deeper on this topic.
What’ s the value Data science application can bring here? Following are some areas where we can apply data science/ML concepts.
Test scoping– Do you need to test all the test cases that are available? Is there a way you can optimize the scope without impacting the quality of the tests?
You can easily apply multiclass classification algorithm to categorize your tests cases into various categories like High, Medium, and Low impact based on the historical data
In Classification, programs are trained from the given dataset or observations and then classifies new observation into several classes or groups.
Bug prediction-Defects identified by customers are costly. How can we identify/predict modules which are defects prone in the earlier stages of test cycle? Defects identified at the customer end is estimated to be 3 times costlier than an internally identified defect.
Develop an automated defects prediction system based on the historical data using ML/AI which will identify the defect prone modules/components. Once the model is built, one can use confusion matrix to evaluate the model for recall and precision
Input dataset can contain no of internal/ customer bugs in the past, no of lines of code impacted, no of testers participated, no of objects touched and other relevant metrics data etc. Now more focus could be given to these identified modules and most likely these areas will generate the maximum code issues for the product.
Incoming customer bug management- Processing huge amount of customer defects is an uphill task. While a lot of manpower is spent on the first level customer support itself, we have a way-out using Data science applications here. A pretrained ML model can identify the code objects and the code fixes released to the customers which are related to the identified issue by the customer. And this will have huge time savings as the first level support engineer need not spend time digging and identifying the root causes of the issues.
Self-healing of automates – As you know applications that are tested are constantly evolving and changing. UI Object property can change frequently, and it leads to the breakdown of the test scripts that are running. A self-healing UI automation tool can adapt the changes in UI attributes like ID, Xpath etc. using a combination of NLP and ML application. This saves huge maintenance time required for automated script maintenance.
Automatic categorization of test failures: -Automated test can fail due to functional issues, data missing, authorization and configuration issues etc. In each test suite which might have thousands of test cases running manually analyzing these failures is cumbersome. Solution is to auto analyze the test results and categorize the failure reasons using ML. Text ML services could be built based on RNN classification and each failure reason could be automatically classified based on the texts in the log files.
Test Project Management– Today businesses must really focus on the efficiency and effectiveness in the resource utilization. These resources (Time, Money etc.) are precious and optimum utilization of those resources would have a high degree of impact on the profitability of the organization which in turn help in maintaining the competitive advantage. Application of ML would help in improving the process blocks bringing down the cycle time and hence the optimization of the resources. This will help in removing the risk and mistakes
Software testers can generate synthetic data for performance and functional tests. And NLP is another technique used to generate test cases. You can explore further and find several other areas within software quality and test management where Data Science and ML can play a huge role.
Title picture: freepik.com