AI in Drug Discovery
3AI October 19, 2022
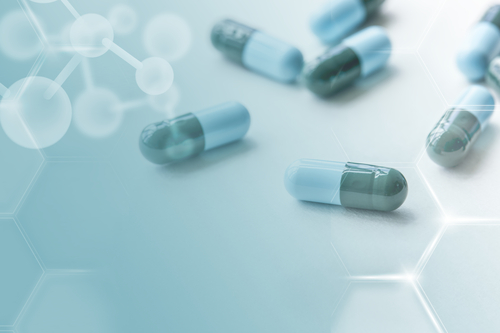
Featured Article:
Author: Vijay Morampudi, Director & Head of AI, Wavelabs Technologies
Drug discovery takes 12-15 years and a median investment of nearly $1 billion to bring a new drug to market. While the true cost of research and development may be as high as $2.5 billion per marketed therapy, when factoring in abandoned trials and clinical failures
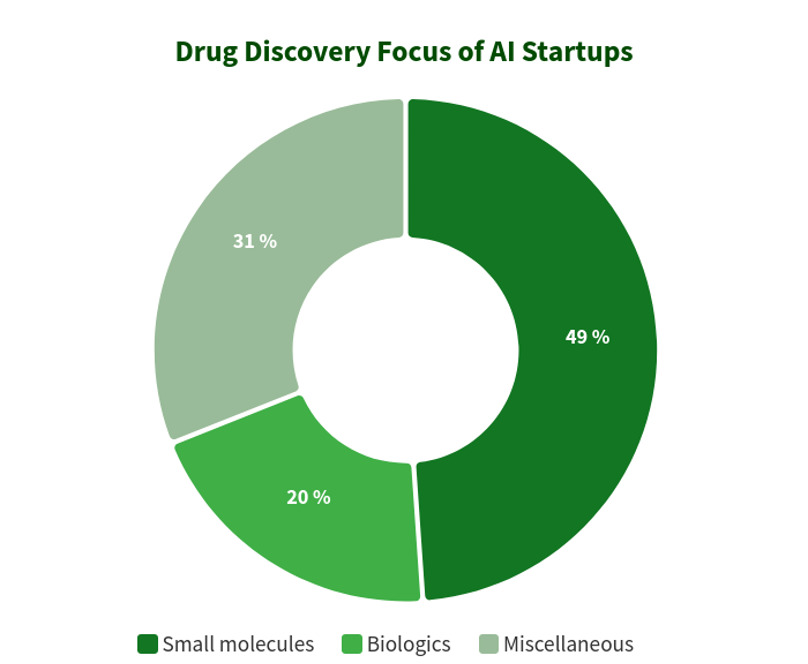
AI start-ups made waves in the drug discovery to reduce time and money spent to bring new drug to market
50% of AI start-ups are focused on small molecules. In today’s drug market, small molecules constitute as much as 90% of global sales
20% of AI start-ups are involved in discovering/developing biologics drugs (antibodies, vaccines etc)
Biotech companies using an AI-first approach have more than have more than 150 small-molecule drugs in discovery and more than 15 already in clinical trials. This AI-fueled pipeline has been expanding at an annual rate of almost 40%.
The global artificial intelligence in drug discovery market size is expected to reach USD 9.1 billion by 2030. It is expected to expand at a CAGR of 29.4% from 2022 to 2030
In this article we look at how AI is applied to Drug Discovery
- Benefits to Pharma Ecosystem Players from Leveraging AI in Drug Discovery
- Finding the Right Biological Targets
- Identifying and designing small molecules for preclinical candidates
- Clinical trials to validate efficacy and safety
Benefits to Pharma Ecosystem Players from leveraging AI in Drug Discovery
A modest improvements in drug development success rates enabled by AI could benefit many players in Pharma ecosystem
Biopharma Companies – Additional 50 novel therapies over a 10-year period, which could translate to a more than $50 billion opportunity. 20% to 40% reduction in costs for preclinical development and 15% increase of approved therapies demonstrating the potential for biotech’s to generate new revenue while helping more patients
AI Pharma Startups – An AI drug development platform could generate significant revenue growth through partnerships, assuming modest annual increases to AI investment within biopharma research and development budgets. With access to years of biopharma experimental data, early-stage AI startups can leverage to further refine their algorithms
Patients – Treatment to patients who are suffering from hard-to-treat diseases
Payers – Trials can generate better outcomes. They can also deliver sizable cost savings by enabling earlier identification and treatment of higher-risk patients
Drug discovery is all about finding 1) a biological target and 2) a drug that acts on the target 3) clinical trials to validate the efficacy and safety
Finding the right biological targets
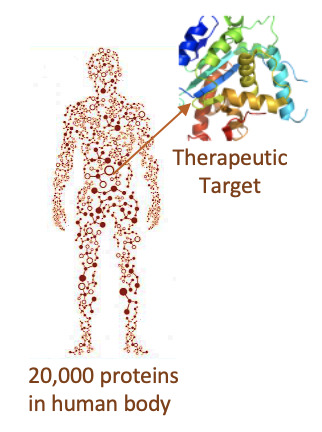
Drug the Undruggable – Of the roughly 20 thousand proteins encoded in the human genome, only a little over 750 are targeted by FDA-approved small molecule drugs. About 2-3 thousand have a small molecule or a biologic under development. Crawl through medical literature, patents and clinical trial databases and use NLP or knowledge graphs to search for disease causing protein. After identifying target a relevant question to ask is does inhibiting or activating this target cause a favourable change in disease activity?
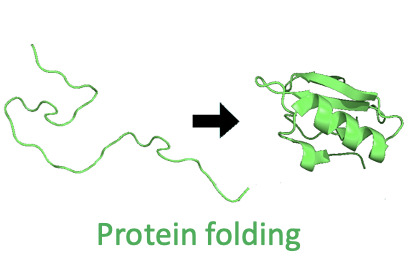
Protein folding – discovering the 3D structure of proteins allows us to find out which compounds can interact with them and how, enabling us to develop new, highly specialised and effective treatments. The challenge is the number of different configurations that a protein might fold into based on its amino acid sequence is astronomical. Any given protein can theoretically adopt something like 10^300 different configurations. DeepMind advanced the understanding of proteins, the building blocks of life, using a transformer called AlphaFold2. It processed amino acid chains like text strings to set a new watermark for describing how proteins fold, work that could speed drug discovery
Identifying and designing small molecules for preclinical candidates
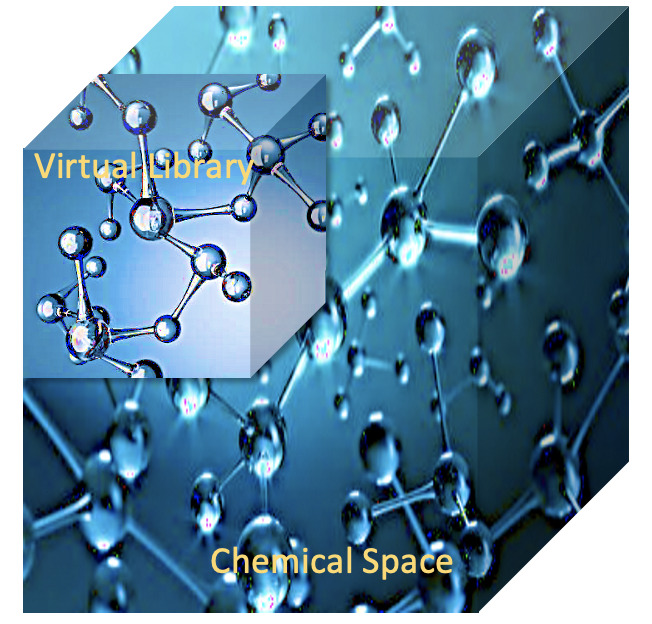
Virtual screening – With 10 to power of 60 theoretically existing drug-like small molecule compounds, the chemical space is incredibly vast. Virtual compound libraries in the range of 10 to power of 9 molecules has become routine. AI can reduce the time it takes to synthesise and screen new drugs by 40 -50 %, reducing costs in those parts of the research process by as much as $26 billion annually
Structure-based virtual screening (VS) can be used to assist in hit identification (identification of active drug candidates). Due to the importance of spatial configurations for physical interactions, determination of binding can be reframed as a computer vision problem. Docked protein–ligand structures are fed into a grid format of protein and ligand channels to act as the input for the model
Drug target interaction – Given data on protein characteristics, use graph neural networks to predict which molecules have the highest binding affinity
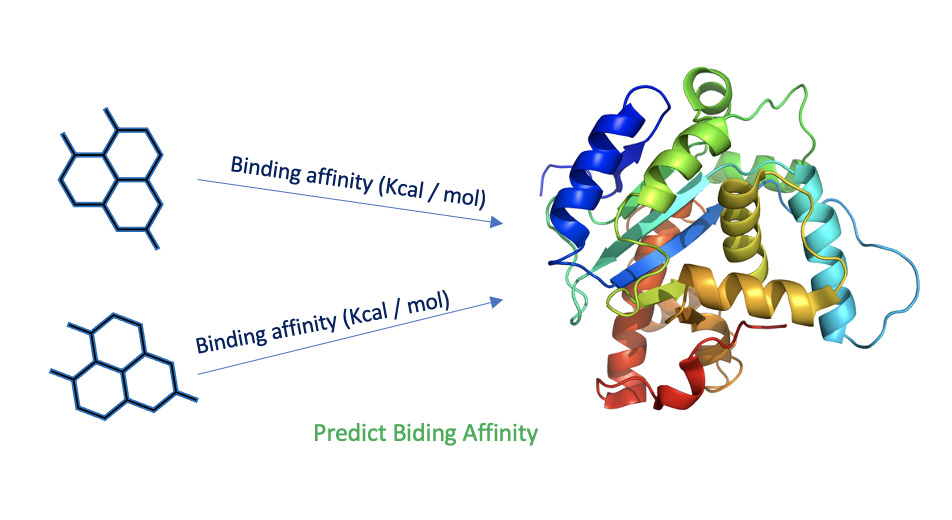
Optimal molecule generator – Use generative and reinforcement learning algorithms to find an optimal solution in line with the constraints of your project.
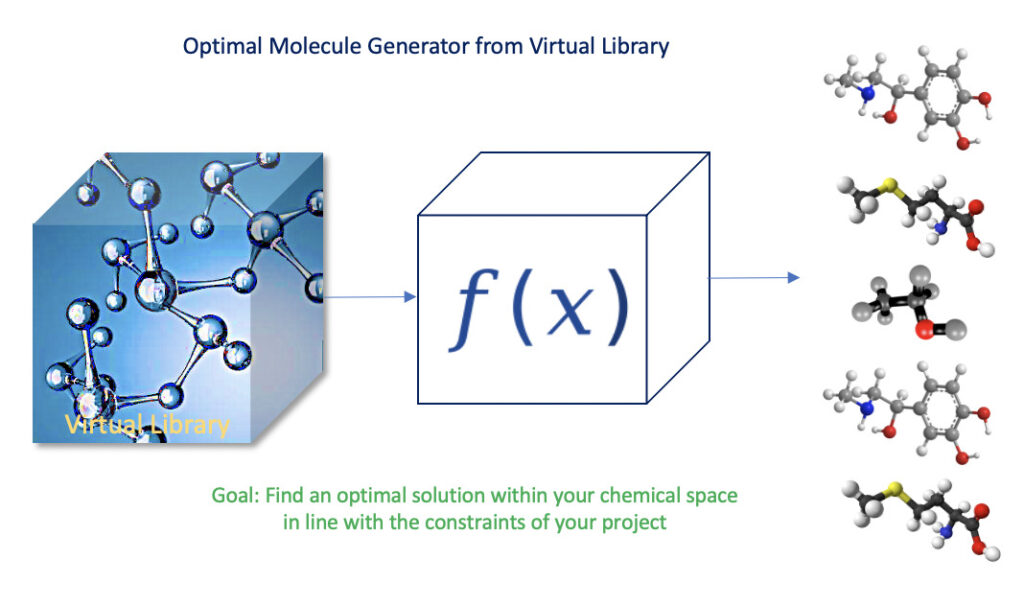
Novel molecule generator – Use generative algorithms to generate new molecules with high novelty, useful for re-scaffolding / new hit discovery
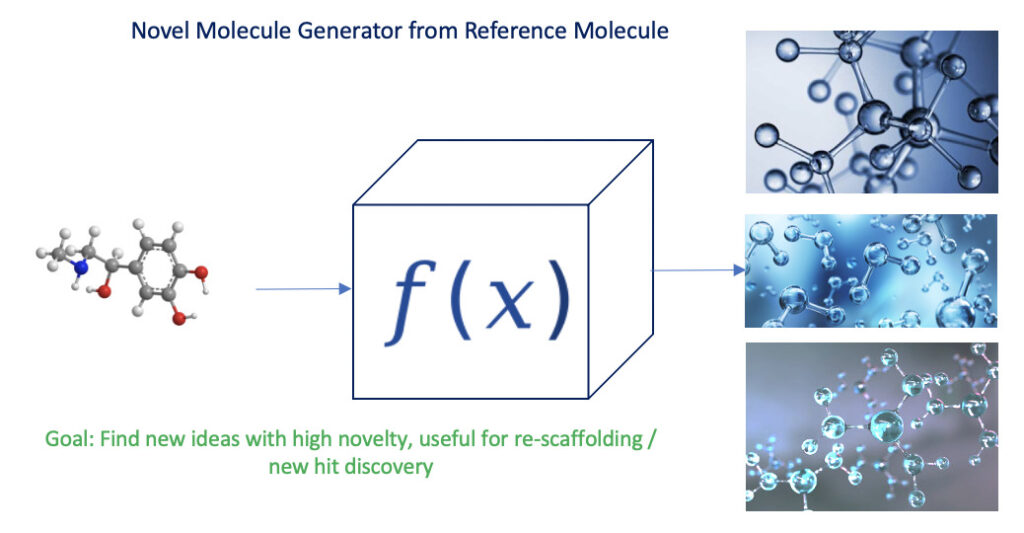
Predict potential drug properties – It is estimated that over 80% of new drug compounds fail during drug development because of poor ADMET (absorption, distribution, metabolism, excretion, and toxicity) properties such as absorption, distribution, metabolism, excretion, and toxicity. A high-quality drug candidate should not only have sufficient efficacy against the therapeutic target, but also show appropriate ADMET properties at a therapeutic dose. Use graph neural networks to predict ADMET properties of a drug
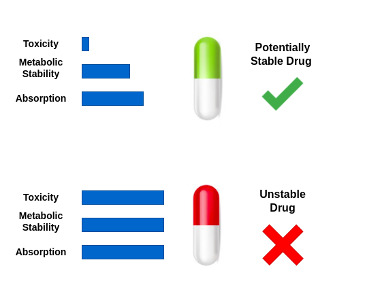
Predict off-target interaction – compute the probability of any drug compound interacting with human off-target proteins. When a drug compound shows a low degree of interaction, it means that it interacts with a few proteins only. On the other hand, a high degree of interaction means that the drug displays activity with many proteins within the body
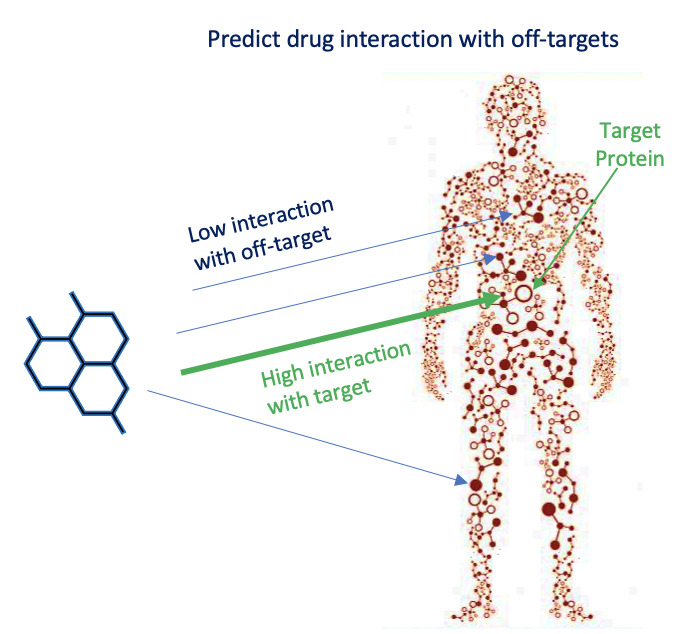
Clinical trials
Clinical trials form the foundation of drug discovery and development. By providing a rigorous scientific evaluation of the safety and effectiveness of new treatments, they allow us to determine whether a potential medicine is ready for use in patients
Patient trial match – Find qualified patients for clinical trials given longitudinal patient electronic health records (EHR) data and trial eligibility criteria
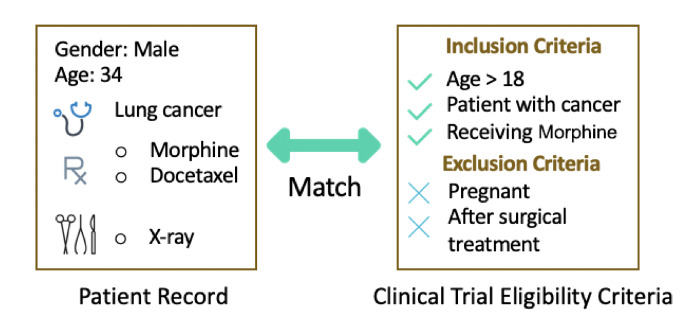
AI to analyse patient records and real-world evidence to match patients with trials. AI-based recommendation has a potential to increase the enrollment rate up to 20%
Predict clinical trial operational efficiency – Around 30% of patients drop out during a clinical trial. At the same time, 40% are non-adherent after few months, which incurs additional costs for the Pharma industry
Trial designers have historically relied on investigator expertise and legacy norms established within sponsor companies to improve operational efficiency while achieving study goals. Use AI to predict clinical trial operational efficiency (dropout ratio, trail duration). These insights to are helpful to trial designers on the potential impact of their decisions on patient recruitment success and trial duration
Predict the chances of a phase-to-phase transition for disease specific trials – One of the main stumbling blocks in the drug development pipeline is the high failure rate of clinical trials. Less than one third of all Phase II compounds advance to Phase III. More than one third of all Phase III compounds fail to advance to approval. Use AI to estimate the chances of a successful phase-to-phase transition for disease-specific trials
References
Revealing the Human Proteome – https://www.nih.gov/news-events/nih-research-matters/revealing-human-proteome
AI Could Speed up Drug Discovery – https://www.morganstanley.com/ideas/ai-drug-discovery
AI in small-molecule drug discovery: a coming wave? – https://www.nature.com/articles/d41573-022-00025-1
Will Biologics Surpass Small Molecules In The Pharmaceutical Race? – https://www.biopharmatrend.com/post/67-will-small-molecules-sustain-pharmaceutical-race-with-biologics/
Drug the Undruggable – https://www.atomwise.com/drug-the-undruggable/
AlphaFold Is The Most Important Achievement In AI—Ever https://www.forbes.com/sites/robtoews/2021/10/03/alphafold-is-the-most-important-achievement-in-ai-ever/?sh=570ed96e6e0a
Virtual Screening – https://pubs.acs.org/doi/full/10.1021/acs.jcim.8b00350
Prediction of Clinical Trial Operational Efficiency https://link.springer.com/article/10.1208/s12248-022-00703-3
Patient Trial Matching – https://arxiv.org/pdf/2006.08765.pdf
Title picture source: freepik.com