Digital Twins: A Way Forward To Unlock the Pivotal Hidden Signals To Increase ROI in Drug Discovery
3AI July 18, 2020
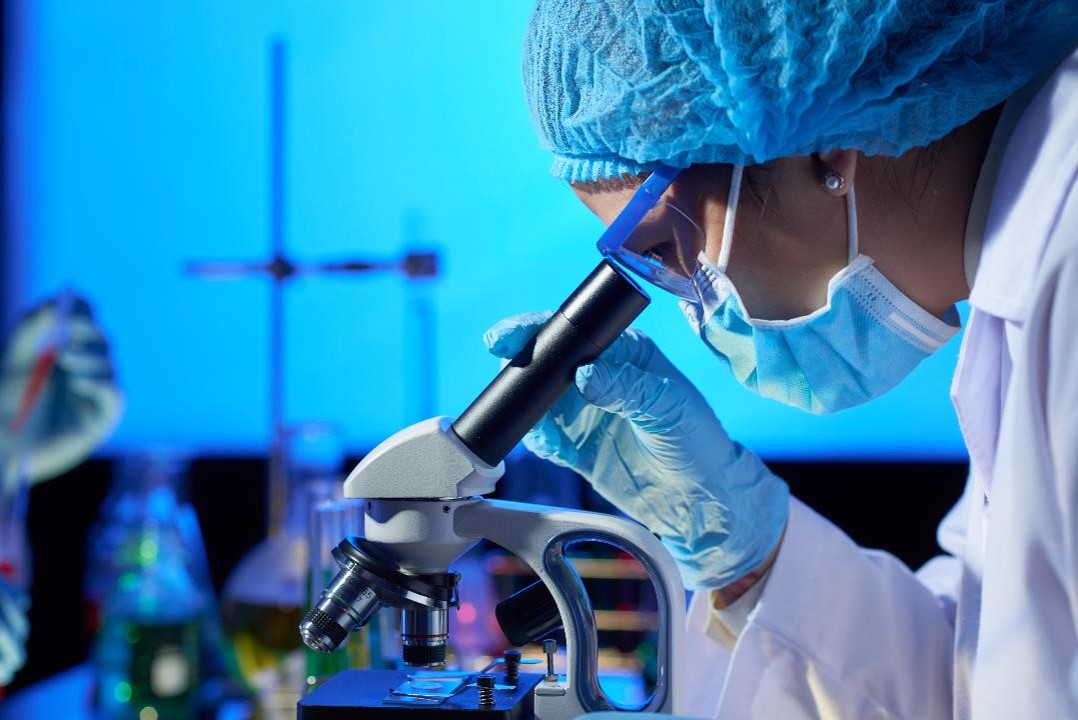
A “Digital Twin” is a virtual or digital copy of a real-world product or living entity (at the atomic or macro geometric level), developed by integrating virtual blueprints with real world data, and optimized through Artificial Intelligence and Advanced Analytics software. The “Digital Twin” concept was introduced by Michael Grieves in 2003. Currently, Digital Twins are implemented in various manufacturing industries, predominantly in aerospace, automobiles, and space technology.
Industry 4.0 (or the trend towards automation and data exchange in manufacturing in the fourth industrial revolution) has enabled large-scale connectivity between the physical and the digital world. A data-rich healthcare industry (where the volume of data has increased by 48 percent from 153 exabytes in 2013 to 2314 exabytes in 2020) together with advances in Artificial Intelligence, visualizations and advanced software analytics, and future quantum computing, is paving the way to creating identical virtual copies of physical entities, ranging from molecules all the way to the complex organs, tissues, and physiological systems of the human body.
This article evaluates the lack of robustness in current drug development processes, from pre-clinical to late phase, and how Digital Twin models can bring sophistication to unlocking the hidden signals and to increase Return on Investment (ROI) in pharmaceutical R&D.
Primary Cause of Late-Stage Clinical Trials Failure
According to the European Center for Pharmaceutical Medicine, the failure of late-phase clinical trials is driven by a variety of factors, including:
- Beneficial effects seen in animal models were not replicated in humans,
- Poor understanding of disease,
- Poor definition of patient populations between phase 2 and 3,
- Phase 2 endpoints were not confirmed by phase III clinical outcomes,
- Insufficient sample size,
- Inadequate dosing in phase 2 and poor therapeutic indices,
- Phase 2 false positive effects were not replicated in phase 3,
- Data-related issues and unintentional unblinding,
- GCP violations,
- Recruitment drop-out and noncompliance with protocol, and
- Insufficient landscape assessment of current standard of care and precedents.
Digital Models and Mathematical Models
Because so many of the trials are in oncology, much of the failure is seen in oncology trials. This article therefore presents a theoretical argument in favor of using digital models to augment mathematical models of tumors.
Currently available mathematical models lack details and are not powerful enough to predict the specificity and sensitivity of drug molecules over individual tumor components, including blood vessels and cellular components, such as biochemical pathways transmitting growth factor signals. Let’s take a brain tumor as an example and evaluate the possible advantage of Digital Twin models: although there are numerous small-molecule kinase inhibitors whose primary targets are of interest for neuro-oncology (e.g., epidermal growth factor receptors, vascular endothelial growth factor receptor inhibitors), there is a no approved drug for such inhibitors to treat brain tumors. This is primarily due to the Blood Brain Barrier (BBB) that prevents certain drug molecules from crossing into the brain and reaching target tumors.
Constructing a Digital Twin model of the BBB based on research data and the BBB properties (such as membrane structure, permeability, lipid and ionic structure, protein folding, three-dimensional structure of receptor drug targets) can overcome this obstacle. A Digital Twin BBB could be evaluated virtually to understand the channels that facilitate diffusion of small-molecule kinases to reach targets, such as the channels’ structure and ionic properties, factors that affect membrane permeability, and the proper molecule orientation. Based on this virtual model, a drug’s target sites could then be modified to allow crossing the BBB effectively and reaching targets.
Digital Twin Models for Preclinical Testing
Among the most cited animal studies in the journals Nature and Cell, only 37 percent of the studies that were replicated in subsequent human randomized clinical trials showed the same results; 18 percent were contradicted in human trials. Another study showed that the discordance between animal and human studies may be due to bias or to the failure of animal models to mimic clinical disease adequately. Although in silico clinical trials testing the drugs in virtual models before testing them in actual subjects improve safety and efficacy evaluations, complete simulations of clinical trials are not feasible with current technology. There is also often an inadequate understanding of the comparative biology between animal models and humans. However, with large amounts of data generated from molecule screening to animal testing, advanced software analytics, artificial intelligence, and three-dimensional modelling, it would be possible to virtually develop the structure of a molecule and its behavior on Digital Twins of animal models. For early-phase researchers involved in drug development this will provide a data-driven visual insight in three-dimensional space in lieu of analytical results expressed in numbers.
Digital Twins for Phase 2 to Late Phase
Digital Twins can provide better insight into and help understand and validate the correlation between surrogate endpoints (biomarkers) and clinical outcomes. An inadequate understanding of biomarkers and the corresponding clinical outcomes results in misleading information about the actual effect of an intervention on clinical efficacy. This is one of the reasons for false positives or false negatives and clinical trial failure. In oncology trials, tumor shrinkage following therapy increases survival. However, the exact nature of tumor shrinkage and how it relates to survival rate is difficult to establish, because the evidence on correlation does not tell us anything about causality. For example, it is unclear if the antitumor intervention or the treatment-induced tumor response affected the patient’s immunological response and survival. A Digital Twin of the patient’s genomic and phenotypic data before and after treatment could be used to answer this question.
Considering the risk of false positives and false negatives associated with biomarkers, it’s important to understand the scientific correlation between the biomarker and corresponding clinical outcome to know whether a biomarker is reliable. A three-arm trial studying pertussis vaccines in Sweden provides a good example of a false positive case: Subjects received a two-component acellular DTP vaccine, a five-component acellular vaccine, or a placebo. The five-component acellular vaccine reduced pertussis in 85 percent of the cases, while the two-component vaccine only provided 58 percent reduction. When comparing the two vaccines, although the five-component vaccine had overall superior efficacy, the two-component vaccine had superior effects on two major biomarkers, favoring the two-component vaccine. It is argued that the misleading results on the relative efficacy of these two vaccines is likely due to the additional antigens in the five-component vaccine that triggered the increased immune response.
Creating data-driven Digital Twins of the vaccines with real-time data (using, for example, multivalent epitope structure, surface protein structure, and cell receptors, etc.) and of the clinical trial subject (considering the subject’s genetic profile and immunological response, such as antibody type, cytokines, interleukin response, antigen and antibody complex formation, and its impact at cellular level etc.) can provide visualized insights into antigens and their effect on immune cells. This in turn helps us better understand the scientific correlation between biomarkers and the corresponding immunological and clinical outcomes and ultimately reduce false-positive errors in Clinical Trials.
Potential false negative clinical outcomes could be avoided by understanding and developing digital models from data obtained from measuring biomarkers and from clinical pathways. For example, in a few instances, the biomarker pathway will have no impact, while the intervention affects the overall clinical pathway and improves clinical outcome.
Visualizing Through Digital Twin Models
Developing Digital Twins requires the seamless flow of data (data thread) and advanced software analytics along with Artificial Intelligence. Based on the successful implementation of Digital Twins in aviation and other electronic manufacturing industries, the use of Digital Twins in drug development shouldn’t be too far in the future. Although possible in theory, implementing Digital Twin models requires extensive inter-disciplinary collaboration on the collection of quality data, integrating data from various sources, the security of data, and the development of mathematical and virtual models and advanced computer simulations. Digital Twins are not likely to replace the existing drug development process, but they may enhance clinical trials and enable candidate molecules to go from preclinical to late phase clinical tests in a sophisticated data-driven approach, and—ultimately—increase ROI. The article is dedicated to the late Clayton Christenson (“Clay”), Kim B. Clark Professor, Harvard Business School. His theory on disruptive innovation made significant a breakthrough in the business world.
Disclaimer: The opinions expressed in the paper are solely of the author do not represent the views of 3AI. This article is being republished here with author’s consent. This article was originally published in https://globalforum.diaglobal.org/issue/december-2019
NOTE: The cover has been designed using resources from Freepik.com