HR makeover enabled by Data Sciences: Talent Sciences
3AI December 3, 2020
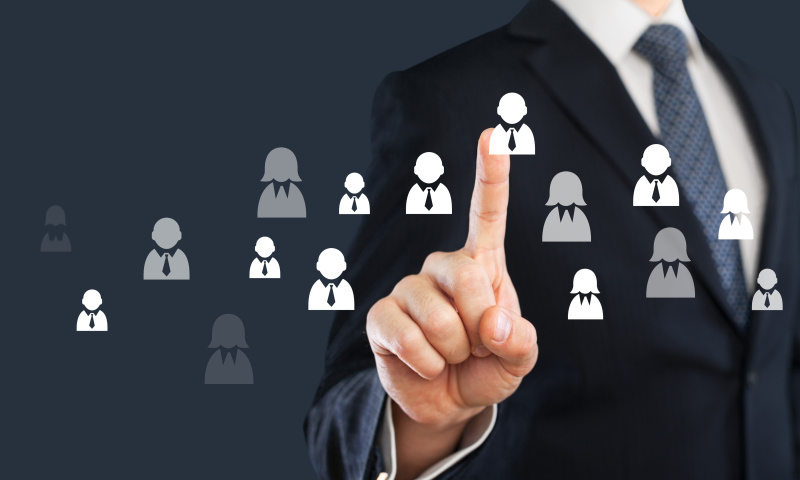
Talent Science is the business capability of using advanced data analysis techniques and predictive models to drive HCM decision making. It calls for a logical connection between decisions about people, HR program investments and strategic business outcomes. This can form a backbone of human resource function of all organizations independent of the sector in which it operates. HR metrics also known as talent science or peoples’ metrics is a sophisticated application of data mining and data science techniques applied on people related data. It is an essential way to quantitatively gauge the spend and the outcome of employee engagement programs and HR systems to measure the effectiveness of various HR initiatives. They provide the companies with the power to measure year on year comparisons on various parameters.
Talent Science enables the company with powerful insights to effectively manage employees to reach the business goal quickly and with high productivity. Challenges abound in this field as to identify what kind of data need to be captured, stored and processed and how to build the model and predict capabilities to maximize return on investment spent on its human resources. Talent Science can be implemented in the following strategic HR and business avenues:
Talent Management
Talent management is a soft space. Historically, we haven’t been able to measure definitely the things that we intuitively believe to be true. But businesses are mandating it. HR is being held accountable to deliver business results. And the language of the business is data science. The growing importance of sophisticated Data Science interventions to HR–not simply reporting what already exists in an organization but predicting what could or should be–is a result of “the recognition that the efficient use of labor and deployment of resources is critically important to the business results of the company”.
The goal is to create a model capable of predicting possible career development based on an individual’s previous experience, industry expertise, education, professional skills, etc. The modeling process requires an extensive array of data to ensure a solid base for analysis, including publicly available vacancies, resumes, recruiting requirements, job descriptions and much more. Also, analyzing an employee’s LinkedIn job profile, the solution provides thorough evaluation of individual talent characteristics, including industry experience, skills and competence to define the factors that are more and less important for a particular career model.
With all this information available, it is easy to create a complete picture of a person’s professional expertise and further compare it against similar available job descriptions, focusing on the career path. This way we can discover not only the best matching positions for every level of competence but also the skills and experience that are required for an expert to move up the career ladder.
Attrition Management Through Prediction
The opportunities to add value through HR practices come more from stopping the wrong outcome from happening, than from reporting on what has happened. For example, the cost of voluntary turnover has been established at approximately 1.5 times annual base pay for salaried employees (Source: PWC Saratoga and CEB). Therefore, if you prevent two high value employees, with salaries of 50,000GBP, from leaving the organization you have saved approximately 150,000GBP. In order to achieve this type of saving you need to know who will leave, before they have left.
This is where sophisticated algorithms, that use historical data to determine the likelihood that someone will resign, come into play. There are a number of known actions that will prevent someone from leaving like signing bonuses, formal agreements around career progression and learning opportunities. However, the crucial part is knowing to whom you should offer these incentives. When it is possible to focus on the right population, through powerful and validated statistical models, it leads to better outcomes for lower cost.
In addition, companies are using clustering algorithms to determine the common features of employees that are related to higher or lower retention rates. This insight means the right approach can be taken with the right employees, leading to better results at a lower overall cost.
Training
Talent Science can also inform training and certification strategies. If the data shows that specific skills are in extremely high demand, recruiters can advise the business when it might be beneficial to invest in training resources instead of seeking out new hires. Rather than spinning wheels in an environment where hiring is especially difficult and time-consuming, training existing employees may be the more economical or timely approach to acquiring the needed skills.
Learning Analytics, a sub-aspect of Data Science can inform the development of every aspect of employee education—including understanding employees, building better programs, and having more insight into the interaction between employees and the software. It allows companies to keep track of learning processes and gauge what is working in employee training and what is not. two areas management can focus on to determine training effectiveness. An internal matrix used to measure employee comprehension can be developed, with key benchmarks and targets. For example, are employees continuing to struggle with certain learning modules? Do they continue to fail certain quizzes or miss certain questions? Second is evaluating engagement—the measurement of whether or not employees are interacting with the content.
Authors of e-learning courses to receive anonymous feedback from learners. Data on individual exercises and on overall courses will provide developers with important insights into how learners use the course. This will help reveal what is successful as well as recurring mistakes and misunderstandings, allowing authors to fix problems and improve courses.
Compensation – Pay for Performance
There has been a steady and constant shift towards ensuring that what employees are paid is closely tied to their contribution to the organization. Some organizations are removing the artificial limits that kept high performers from earning more than their managers. For a number of the companies we work with the alignment of pay for performance is the number one shared agenda item between the CEO and the head of HR.
One of the best ways to demonstrate this practice is through a metric called the “Performance based compensation differential”. This metric expresses how much more high performers are paid compared to their average performing peers. For example, a score of 1.2 means that on average high performers receive 20% more compensation than average performers. Turning this critical question into a single number allows for powerful insight across the organization; it means that different locations, business units and groups of employees can be easily compared using simple visual analyses.
One powerful way this translates to business value is during the annual pay review cycle. Most HRIS systems allow you to enter changes in pay, however these systems do not enable you to analyse how these awards relate to performance and whether or not they are aligned to the goals of the organization. Most HR departments provide guidance and then trust their managers to get it right.
Being able to analyse all of these decisions in real time, report this back to the organizational leadership and then revise these adjustments before they are confirmed leads to a demonstrated ability to ensure that the budget increases going into labour costs are being applied in the optimal way.
Retirement
Another place where prediction is becoming valuable and important for the companies we work with is in relation to retirement. The pattern of behavior relating to retirement is changing with more and more people delaying retirement or shifting to contract or part-time work than ever before. Prediction here is important as often the people retiring are in key roles or hold key relationships and are critical for the business to ensure continuity of performance. However, it is also challenging to keep a potential successor waiting if the incumbent chooses not to retire at the time expected.
Instead of using the old indicators of age and tenure to estimate retirement behavior modern data science applies algorithms that take into account many additional factors such as recent changes in role, pay level, rates of change in pay and incentive eligibility to refine the prediction of who will retire. This allows companies using this type of analytic approach to be more successful and effective in managing the retirement cycle and ensuring that key roles have a successor ready at the right time.
Sales performance and recruiting
Many companies have analyzed the profiles of top salespeople and now know that screening candidates for grade point average or academic pedigree is no longer considered a strong indicator of future sales performance. Companies are turning to data science to get an eagle-eye view of their inherent employees and assessing their competency levels and creating a customized recruiting trend which does away with hiring under-performers. A high-tech company developed an data science model that accurately predicts job candidates who are likely to become “toxic employees” (those who lie, cheat, or commit crimes) and dramatically reduced this population among its hires by scrutinizing special parts of the interview process.
In today’s tight talent market, understanding both the local and macro conditions that may impact talent availability, salary and benefit decisions, and hiring timelines helps recruiters set expectations and adjust their approaches to attract candidates for hard-to-fill positions. Are other companies in the area currently on the hunt for similar skills? Is the salary being offered lower or higher than average? Is the skill set needed easier to find in a different geographic area? Data captured from job boards and other sources of information on talent supply and demand can help answer these questions and give recruiters the ammunition they need to get business buy-in on key decisions related to hiring strategy. Armed with data, recruiters’ discussions with hiring managers change: recruiters build immediate credibility and transform their roles from advisors to decision makers.
Productivity Spike
Software companies, retail banks, and manufacturers are looking at the characteristics of top salespeople, realizing that their personal networks, how they work internally, and the time they spend with customers predict results much more accurately than the amount of sales training or experience. IT and HR departments are now looking at email metadata to understand why some people are more productive than others, then reducing the number of internal meetings to improve output.
There are some companies who are studying the patterns of unplanned absences to predict when people are likely to take a day off, pre-scheduling extra staff to make up for known periods of absence. This makes sure the total utilization doesn’t vary strongly.
Data science is even being used to analyze the correlation of productivity to ambience or working conditions / environments. Companies like Deloitte Canada are experimenting with smart badges, using them to gather data suggesting that offices with larger shared work rooms, more light, and more inter-company collaboration have higher productivity.
Compliance & Risk
A lot of systematic and unsystematic information relevant to employees is available to the HR which can be leveraged to create, measure and redesign the policies and assess their compliance levels by employees. Sentiment analysis involves more than just the annual surveys. The data should be continuously tracked, analyzed and scrutinized on key topics. External data like Facebook, Twitter, LinkedIn etc. provide valuable feed for sentiment analysis.
Talent Science minimize the threat of internal fraudulent practices from employees through non-compliance by identifying and/or predicting employees at high risk to violate security policies or other company regulations. Once the employees risk is assessed proactive actions could be taken for corrective actions. There are several ways talent science is being implemented in this area. Banks are studying patterns of fraud and noncompliance at employees’ end, and can now predict behaviors that will likely result in unethical behavior. Also, a UK financial services company uses Data Science to evaluate individual employees, spotting potential “rogue traders” and other compliance breaches as a part of proactive risk management.
Building Culture using Data Science
By examining this data in aggregate, for example, one manufacturing company, discovered some of its junior managers spent more than 30 hours every week “managing up” with reports to senior executives or in status meetings. Bottom line: that left just 10 hours of time for “real” work for the host, not to mention the ripple effect across their own teams.
This is the brave new world of talent science which uses time management data to help companies understand the relationships—external and internal—driving corporate decision-making. Once a company understands the behaviors that correlate to success, they can measure them. Many of these organizations use the technology to tame meeting overload. Some also use it to examine how the habits of high-performing sales representatives differ from others. There are real behavior differences between great performers and average at each customer.
It is increasingly becoming mainstream to base the intricacies of organization culture based on data and insights. A team of organizational development experts and data scientists from eBay measures the strength and adoption of its cultural values through a combination of internal and external data metrics. To compare eBay employees’ views with external perspectives, the team also conducts thematic analysis and natural-language-based analysis on news articles and Glassdoor to get a view of the external market perspective of eBay’s culture.