Importance Of Data-Centric AI In Business And The Role Of Observability In It
3AI May 10, 2023
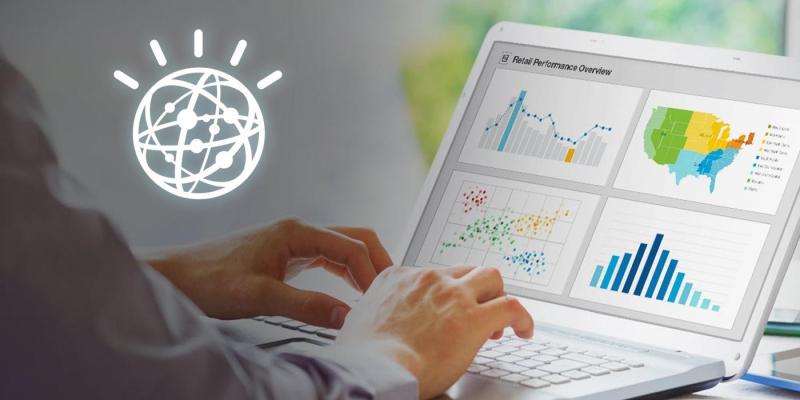
Featured Article:
Author: Anirban Nandi, Vice President, AI Products & Business Analytics, Rakuten India
We all have heard the old saying that “Data is the new oil”. But wait!! Is that completely true? Just like oil, unrefined data is of little to no use. So, allow me to correct the maxim a little – “Quality data is the new oil”.
Challenges in becoming a data-driven organization:
Every day we are creating 2.5 quintillion bytes of data. Just to give an idea of how big that number is, a quintillion is one followed by 18 zeroes. Every average person is creating 1.7 MB of data every second. Almost all organizations in today’s world desire to become data-driven to win over their competitors. Research, development, and application in the field of Analytics, Machine Learning, and Artificial Intelligence are at an all-time high. So, both the will and the required tools are there. But have we reached the full potential of being completely data-driven businesses? According to a report by Harvard Business Review, only 26.5% of organizations have become data-driven. So, what is stopping organizations and their leaders from becoming completely data-driven and take crucial decisions based on data and automated processes? Along with other things like culture, business acceptance, and the uncertain nature of data-driven decisions, using the right data is one of the biggest bottlenecks. According to the same report of Harvard Business Review, “Today, corporations encounter vast new volumes of data, as well as new sources of data, which include sensor data, signals, texts, pictures, and other forms of unstructured data. It has recently been argued that 80% of all new data is unstructured, meaning that it is not easily captured or made quantifiable.”
Need for data-centric AI:
Any AI system consists of two parts. Code/algorithm and data. Throughout history, we have been seeing tremendous development around coding and algorithms. However, the reality is if we feed garbage into algorithms, they will throw out garbage. Feeding even the most advanced algorithms with unrefined or inferior data will lead to sub-optimal or even wrong decisions. That is why Landing AI CEO Andrew NG said “Instead of focusing on the code, companies should focus on developing systematic engineering practices for improving data in ways that are reliable, efficient, and systematic. In other words, companies need to move from a model-centric approach to a data-centric approach”. Hence, collecting, labeling, and continuously monitoring data is extremely important. The more complex the system, the more robust should be the data pipeline.
What is data-centric AI:
Think of a Data-Centric AI system as programming with a focus on data instead of code. Instead of the algorithm, working on the data is the central objective, and rather than gathering more data, more investment is made in data quality tools to work on noisy data.
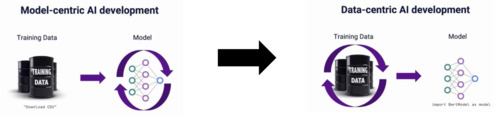
Apart from other benefits, it helps in improving the performance of analytical methods, reducing development time, and developing a consistent analytical pipeline as the uncertain nature and low quality of the data are largely gone, taking organizations one step closer to becoming completely data-driven.
Next, let’s see, what are the challenges in going from a model-centric approach to a data-centric approach.
Data quality and labels:
Often the real-life data and its labels are very different from the data used for analysis or model-building. On the one hand, ambiguous or incomplete labeling of data can lead to wrong output. On the other hand, domain gap, bias, or noise in the data stops us from getting the desired result from the analytics pipeline. Different types of weak/self-supervision and data augmentation often help to solve these problems. Details of these algorithms are out of the scope of this article.
Having said that, both the data quality and labeling cannot always be controlled. Most of the time, organizations can’t have more quality or noise-free data, that is apt for the business, without incurring more costs. However, even in those situations, organizations still can do a couple of things to take the right decision from the existing data. The first one is obvious. Selecting relevant information from existing data, cleaning them properly, and analyzing them manually to remove any anomaly or bias. However, this is more of a manual/semi-automated task done by the analysts or modelers. The second one, however, can be set up at an organizational level and automated to a large extent. This is about collecting and using the data that tracks the performance of the current systems and is fed back into the system itself to enhance it further. This is often done using Observability and Monitoring. These two should be part of any system that is deployed or even being tested, especially in those which depend on data and ML algorithms. Observability and Monitoring alone can help in taking lots of correct data-driven decisions by eliminating the scope of error or sub-optimal performance of systems.
Observability, monitoring, and data-centric AI:
Observability is the property of a system. It is a measure of how well a system’s internal state can be understood from its external outputs. Observability is important to have greater control over any complex system. It enables the user to understand why something is wrong in a system.
Monitoring on the other hand is observing a system’s performance over time through different metrics. It tracks the health of a system.
Both Observability and Monitoring complement each other and go hand in hand. While Monitoring tells when something is wrong in the system, Observability tells the reason. Most complex systems, especially those depending on data and Machine Learning, require continuous use of both. As time progresses and new data comes in, Machine Learning systems start deteriorating and at times fail. Hence, continuously checking the performance, gathering data about the same, keeping track of different metrics, and updating the analytical layer or the model from time to time is extremely important. Observability and Monitoring are thus an integral part of moving toward a data-centric AI approach.
Technical debt and role of observability/monitoring:
Whenever something is developed and deployed quickly for the quick win, we end up incurring future costs due to multiple reasons like lower productivity, rework, or additional operating costs. However, many times, it might be unavoidable due to many reasons. Just like financial debt, technical debt also accumulates over time, and paying it off promptly is extremely important. It becomes even more crucial for an ML/AI system due to the uncertain nature of the algorithm and the data. Observability and Monitoring, both play a key role in estimating technical debt by analyzing past performance, tracking the current state of the system, and taking necessary steps for different system failures or low-quality outputs which, otherwise, can incur and accumulate huge costs over time.
Conclusion:
In today’s highly competitive environment, organizations must become data-driven, and to achieve that, they must take a move toward Data-centric AI, which is the future of analytics. In this article, we explored one of the major problems organizations face in taking important decisions based on data which is the quality of the data itself. We saw how along with other methods, Observability and Monitoring play a crucial role in taking a Data-centric AI approach. These are some of the must-have tools to take better and right business decisions driven by data, have an edge over the competitors, and win the market.
Reference:
https://hbr.org/2022/02/why-becoming-a-data-driven-organization-is-so-hard
https://towardsai.net/p/data-centric-ai/the-principles-of-data-centric-ai-development
Title picture: freepik.com