Reorienting data sciences for banks: The new normal
3AI January 2, 2021
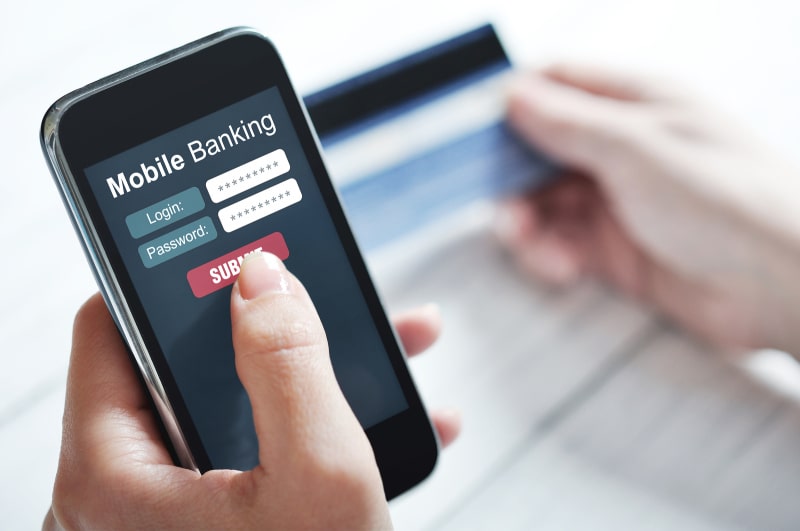
Digital data has snowballed, with the proliferation of the internet, smartphones and other devices. Companies and governments alike recognize the massive potential in using this information – also known as “data detonation – to drive real value for customers, and improve efficiency. Analytics could transform businesses and economies, but the real game changer is data science.
For banks – in an era when banking is becoming commoditized – the mining of banking data provides a massive opportunity to stand out from the competition. Every banking transaction is a nugget of data, so the industry sits on vast stores of information.
By using data science to collect and analyze big data, banks can improve, or reinvent, nearly every aspect of banking. Data science can enable hyper-targeted marketing, optimized transaction processing, personalized wealth management advice and more importantly, risk management.
Data Science can be very valuable for finding patterns and attributes that indicate a higher propensity for fraud. For example, scoring models use attributes from the credit card application to make a judgment about whether a payment should be routed down a different validation path and be subjected to additional research before it is approved or denied altogether. By implementing this capability into systemic processes for decision management analytics – along with the score cuts and flags that are established as a result – financial services organizations can significantly reduce fraudulent activity. Now, through the power of data science, innovators in the industry are leveraging new technology to proactively expose fraud rings’ biggest vulnerabilities – shared identity information. Link analysis is bolstering Big Data’s broad reach, using more information to identify more relationships.
Forecasting Profitability Components – When analytical techniques are applied for vintage financial forecasting, banks can predict such details as delinquency, closure, and charge-off accounts, resulting in more effective product and pricing decisions. Forecasting payments and loss can also be considered a way of forecasting fraud. On the front end, there are the new customers who default on their first payment. Regardless of tenure with the company, the impact on the forecast profitability of accounts is a direct byproduct of delinquency and charge-off loss. It requires extensive human capital and technology to forecast these things. Especially since the Card Act of 2009 squeezed profit margins, credit card issuers have had to be extremely accurate and pay much closer attention to these details in forecasting new product and pricing strategies and understanding the profitability of accounts. Perhaps not surprisingly, generating the right amount and type of data in the right format for analysis will be critical to economic success and demonstrating a return on investment.
Pricing – As banks seek to recalibrate their business models to suit current regulatory and market conditions, one crucial factor in their plans will likely be customer response to retail pricing shifts. Institutions will potentially have to redouble their efforts to engage and win over skeptical customers. They should consider investing in a data-driven understanding of their customers to tailor efforts to strategically important segments such as the Loyalists identified in this survey, as well as attempt to convert Distrusters to Loyalists. Resetting customer expectations may be challenging, but with a targeted approach it may lead to substantial competitive advantage.
Transactional Behavior Analysis – With the emergence of new channels, transactional Behavior Analytics is the key to serve the customers with their preferred way/right channel. Behavior Analytics can help financial institutions analyze transactional behavior aspects (for example – recency, frequency and the monetary value of customer’s transaction and profile). They also help reveal channel preferences and usage for specific products and transaction patterns across customer segments.
Optimizing Check-Float Criterion – Optimizing check float criteria is another piece to the Data Science continuum that can significantly reduce bottom-line costs. Whether one call it outright fraud or not, people will write bad checks in the hopes of circumventing the check floating process. To understand which checks to honor immediately and which to float, some financial services institutions use a purchased, third-party model. Transaction records go through a decision management process where a behavioral scoring technique is applied and score values are generated to help determine whether to float a check and for what period of time. Predictive analytics determine the best parameters for that process.
Collection – In the collections area, propensity to pay has several implications. There will be customers who may have had the best of intentions when they started the relationship, but unfortunately, they don’t always follow through. Scoring models help to optimize call center resources and manage potential fraud by providing decisions as to the proper treatment for a customer. For example, if an account is delinquent, should the customer be given additional time or forgiveness for certain fees? Through a propensity-to-pay model, financial institutions can also spot fraudulent behavior, because it can more clearly identify those who had no intent to pay in the first place. These are customers who were approved on the front end, welcomed into the portfolio, but their behavior indicates that they had no intention of paying, and they’re probably going to roll right on out the door.
Cost Reduction – Data Science help compute operating costs per activity type. The cost can be referred for various combinations of channel, product and customer segments. Customers using live channels (for example, branch, call centers) cost much more to serve than customers using self-service channels. Analytics are very useful in determining channel profitability; banks can design strategies for migrating customers to low cost channels.
Customer Satisfaction – Data Science can help monitor customer satisfaction on service quality, by providing insights on evolving customer needs and satisfaction levels. Customer satisfaction levels on non-financial interactions made with bank can also be monitored using analytics. Building a 360 view of customers by integrating cross-lob and external (third-party/credit bureau data) is important to get a holistic picture of customer demographics, engagement, need and preferences which in turn helps to serve customers their preferred way/increase customer satisfaction.
Customer Retention –Identifying and retaining at-risk affluent customers remains a top priority for bank executives. Data Science play a major role in reducing voluntary customer attrition or increasing customer retention. American Express’s global B2B marketing team is using predictive analytics to identify at-risk customers who otherwise look healthy in its database. Through a pilot project, the company was able to improve identification of attrition risks, and arm their marketing and sales staff with more accurate lists of individuals to target with retention campaigns and communication.
However, many banks have not yet been able to generate actionable insights from available data or effectively integrate data science the way they think and operate. The key is to start small and take manageable steps toward incorporating Big Data analytics into their operating models. It is crucial for all banks to realize the importance of Data Science and act on it —- or they can ignore this at their own peril.