Role of Generative AI in the Realm of Generative AI
3AI March 4, 2024
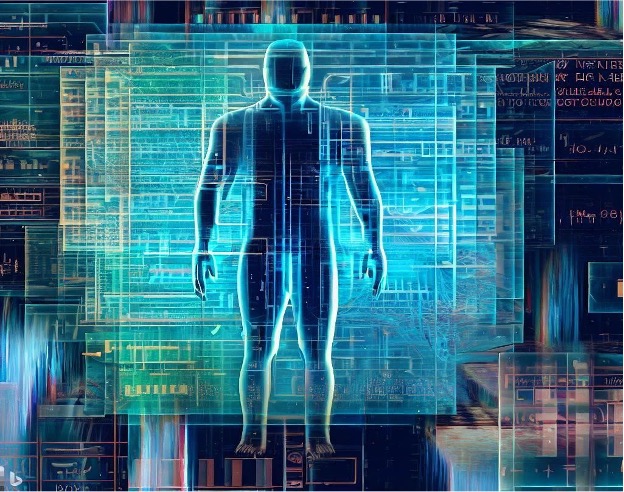
Featured Article:
Author: Ramana Kompally, Director – Data & Analytics, Cloudsufi
Generative Artificial Intelligence (AI) has taken the world by storm and every organization is looking forward to having a pie in the cake and augmenting the portfolio. While a plethora of applications is being envisaged using Generative AI (Gen AI), there is one niche area that is generating a lot of interest and traction in the technological space.
As Humby said, ‘data is the new oil’, the lifeline of an organization and a vital asset for the success of Artificial intelligence. Insights from a large scale of real- time quantifiable and data-driven evidence, delve us to understand the system better, make informed decisions, create newer opportunities, and intelligent products, and improve customer experience, consumer behavior trends, and data strategy, to name a few models to usher growth. There is no dearth of data troves in the enterprises. without compromising the quality and context of the data. This is where the world in engendering data generation mirroring the real data. Synthetic data to the AI world are mind-blowing right from data generation on demand to “by 2030, synthetic data will completely overshadow real data in AI models.”
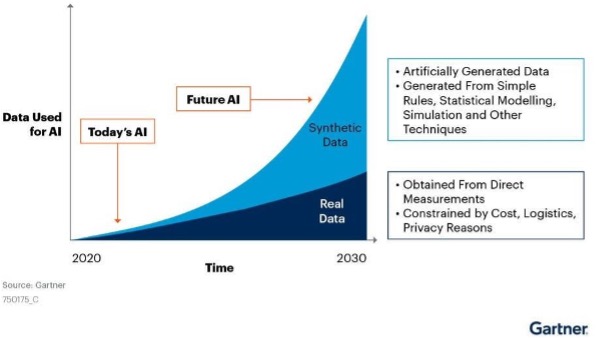
SD can create diverse datasets in large quantities for training Machine learning models reducing the dependency on real-world data and leading the next generation of innovation. According to the Nvidia blog, “Donald B. Rubin, a Harvard statistics professor, was helping branches of the U.S. government sort out issues such as an undercount especially of poor people in a census when he hit upon an idea. He described it in a 1993 paper often cited as “the birth of synthetic data.”
“Each one looks like it could have been created by the same process that created the actual dataset, but none of the datasets reveal any real data — this has a tremendous advantage when studying personal, confidential datasets,” he added. While we will try to delve into the synthetic data applications with special reference to Generative AI at the same time avoid the deep dive into the technical discussion. Today, with the advancement of Gen AI every enterprise is looking to capture the advantage of the technology more specifically the synthetic data for advanced analytics adding new dimensions.
Synthetic data can be generated for tabular, voice, text, Image & Video requirements. Tabular datasets are generated retaining the statical properties and structure as they may contain the information that may show up the identity of the person (PII etc). Therefore, the data is required to be anonymized (removal of characteristics that may identify a person) and synthesized. Some of the popular models that do tabular synthesis are CTGAN, WGAN and WGAN-GP. While tools are already available, the generation of synthetic sound data may help scientists to accurately enhance the defense capabilities by understanding the radar waves, sounds of different missiles, tsunami predictions based on the sound of the waves in different environments, sounds of seismic activity, and text-to- speech. Generated Images and Videos have varied applications from computer vision, face generation, training machine learning models, and simulation of a variety of situations. Synthetic test data is useful for training the chatbots, detecting abuse, summarization of documents, spam check etc
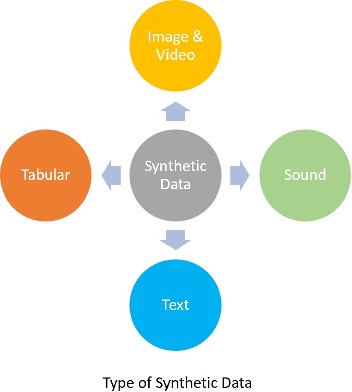
Synthetic Data Generation and its Essence:
The average Chatgpt user will tell you the importance of the tool and its ability to provide answers for questions belonging to different domains. Similarly, someone who has translated their dreamy vision into art using tools such as Midjourney and stable diffusion will present you with various advantages of using the tool. While the medium of data generation only changes, the ability to leverage such tools to solve a problem evolves.
Consider the scenario where an individual is tasked to develop a fake news- detecting bot. He/she ventures out to collect data, curates it and thereafter cleans it. Post training their computational engine or language ‘model’ understands the, the user finds out that the learning system is flawed and has its own bias. The individual goes on to use anaylze what area of the dataset caused the model to behave with such nuances. To solve the same, he/she uses Bard, LLaMa and others LLMs to generate data in the same lines as the missing piece of the puzzle and then puts the model to train. This kind of approach further helps the user and his/her organization to reduce biases, improve accuracy and cater to a large amount of data influx which are the inherent challenges of detecting fake news at scale.
Synthetic Data Generation, like I mentioned previously, has always existed. But, its significance now lies in accelerating the space of Enterprise AI. Synthetic Data can help solve AI biases, address fairness and alignment provided it is controlled and tamed for a particular task or a purpose.
Few years back, Google released Duplex that could book your hotel/restaurant reservation, and this stands as a testimony to use AI powered systems at scale and increase productivity. Generative AI, if fine-tuned or streamlined to a specific task/purpose definitely saves time, money and increases the users overall productivity leading to a number of positive routine changes.
Data Sufficiency being a major concern for startups and enterprises that are developing AI based solutions, the dearth of flawless datasets possess a challenge. Consider a company that works on developing autonomous driving vehicles, where millions of hours of driving data are required for training the algorithms. It would be immensely time-consuming, expensive, and logistically challenging to gather data, curate it and process it for the same. This is where synthetic data comes to the rescue. Using Generative AI, the company can create a range of scenarios and situations within a virtual environment, generating high-quality data that can be used to train and fine-tune their autonomous systems.
Synthetic data also has the potential to overcome privacy and alignment concerns, which often pose a road-block in data-driven fields like healthcare, retail and finance. For instance, medical researchers who need access to patient data and their health records to build predictive models can use synthetic data that imitates the statistical properties of the original data and its other correlations, without revealing sensitive personal information. Similarly, in the finance industry, synthetic data can be used to create realistic data on customer behavior and inherent patterns they project without the risk of compromising privacy regulations. Similar is the case with retail. Personalized customer experience, preferential treatment based on the behavioral data, fraud data, demographic references, targeting a particular location based on the spend analysis, age, gender etc are all now possible with the help of data generated by Gen AI
Generative AI for Synthetic Data Creation:
The magic behind the generation of synthetic data lies in the realm of Generative AI. At the core, these models learn the underlying distribution of the original data and then generate new data points with similar properties. Among these, Generative Adversarial Networks (GANs) have demonstrated remarkable capabilities in creating realistic synthetic data.
GANs operate on a game-theoretic approach where two neural networks, a generator and a discriminator, play a continuous game of deception and detection. The generator network creates new data instances, while the discriminator evaluates them for authenticity. This tug-of-war kind of a game, eventually results in the generation of synthetic data that is very identical and holds the same properties of that of real-world data.
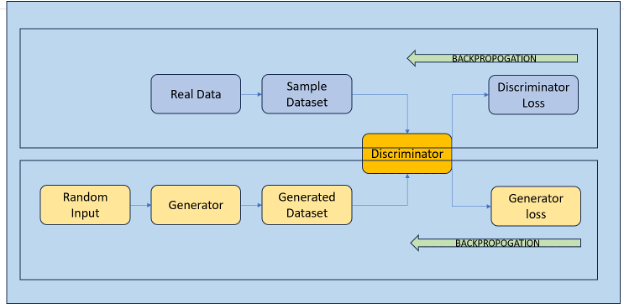
Generative AI use cases for Synthetic data:
In the domain of e-commerce, GANs can be used to create synthetic customers with diverse purchasing behaviors. These synthetic customers can be used to train models that predict purchase behaviors, optimize pricing strategies, or manage inventory, thus allowing companies to test their strategies without the need for extensive real-world data. Google, in the past few days, has released their AI that can allow the user to virtually try out a wide range of clothing. Similar models and use cases have surfaced out in the research community for a while now and the potential for such algorithms is limitless.
In another instance, consider a company trying to optimize its logistics. Real-world logistics data is often erratic and incomplete, presenting a challenge to an organization trying to streamline its operations. Diffusion models, which are probabilistic generative models that mimic the spread of data points in a multi- dimensional space, can be used to accelerate logistics planning and functions. They can generate synthetic data that accurately reflect complex real-world logistics scenarios, enabling the company to experiment with various optimization strategies without risking disruptions in their actual operations.
Imagine, generation of MRI scans and CT scans that resemble the real world aiding the research work in finding the ailments at the initial stages even before they occur. We have seen how the diabetic retinopathy images collected by google helping the physicians in identifying the cardiovascular diseases.
Diffusion models have been in the news for some time now, with the dawn of new age AI art generators and text-2-video generators, these models have proven to generate realistic images and audio as well. While these systems and engines catalyze the ability to support one’s vision, they continue to pose a doubt and raise questions as to how they align in the Ethical and Moral standpoint when made mainstream! Imagen, DALL-E are excellent examples of Gen AI application where images are created based on the textual description. Google has developed Med-PaLM2 specific to the needs of Healthcare and Cyber-PaLM2 for cyber professionals.
Conclusion:
In conclusion, synthetic data offers a powerful tool for organizations to overcome the challenges of data availability, privacy, and quality, and it has the potential to accelerate the adoption and sophistication of enterprise AI. Generative AI, with techniques like GANs ,Diffusion Models and Large Language Models, play a pivotal role in creating synthetic data that is realistic, diverse, and fit for purpose. However, while the promise of synthetic data is vast, it should not be seen as a panacea. Just like with any data-driven approach, it is crucial to ensure the quality and relevance of the synthetic data, and to verify the results with real-world testing. As with all things in AI, a thoughtful, careful, and responsible approach will yield the best results with no harmful side effects.
Title picture: generated by AI