What CIOs can learn from the first 30 days of an AI Copilot
3AI August 21, 2024
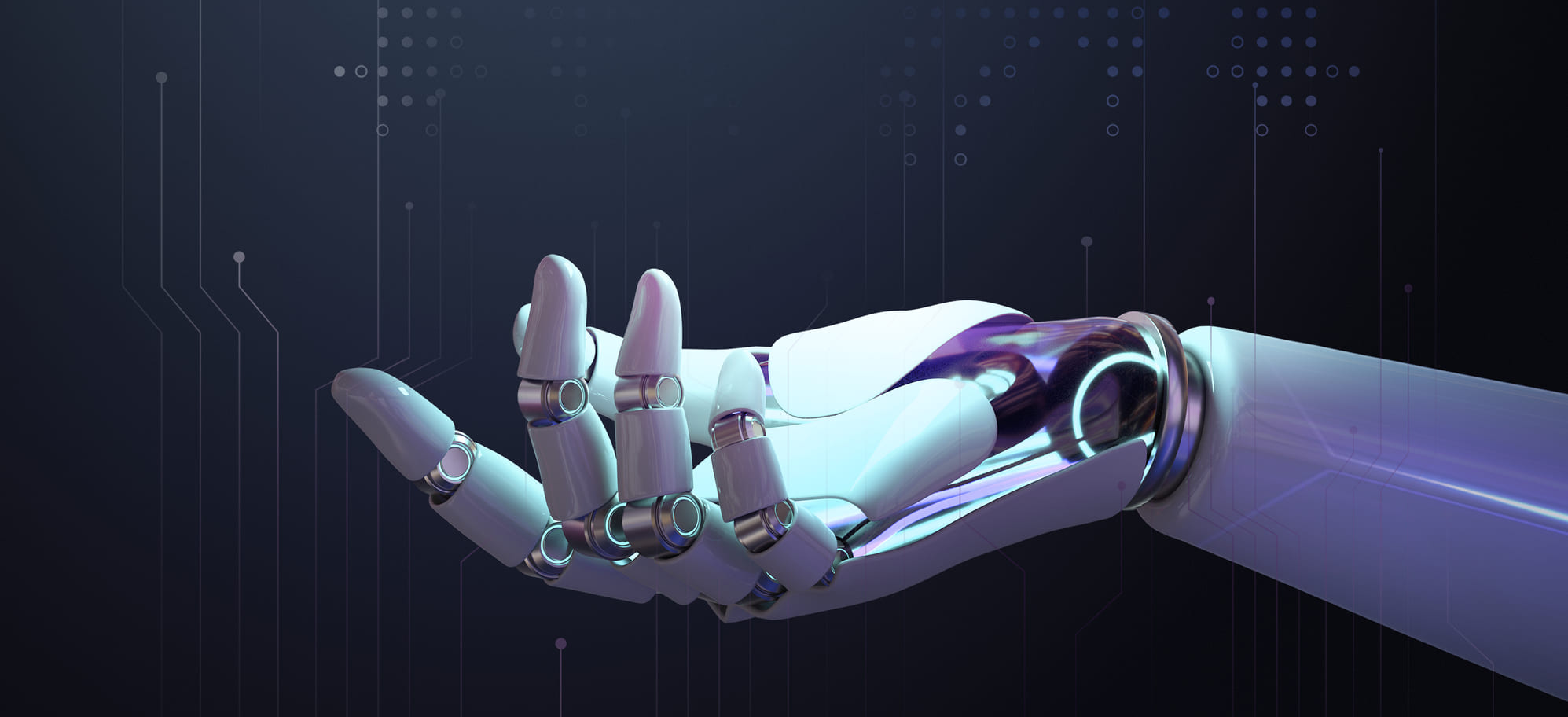
Featured Article
Author: Koyelia Ghosh Roy, EXL
Copilot is a buzzword now with almost all assistive solutions being tagged with them. However, it is the most in-demand solution in today’s era, helping from simple automation of mundane tasks like O365 CoPilot or very sophisticated, customized solutions for specialized tasks like data analysis using database agents and Foundation models. Nevertheless, all of these require meticulous assessment and strategy to be successful.
Hence, to be really successful, below are some of the key learnings that any CIO could take from the first 30 days of an AI CoPilot initiative:
Data Management: Data is the most critical element for AI solutions. The below to be evaluated for an effective Co-pilot solution:
- Data Integration: Integration with data sources, managing the data refresh cycle, and stringent version control are the keys to a reliable, robust solution.
- Data Quality: Datasets accuracy is paramount for reliable outcomes.
- Data Landscape: Identifying the file type, data type, and storage with data purging rules impacts the solution construct.
- Data Governance: Responsible AI plays a very important role. A strong AI governance model must be well framed and implemented, ranging from model evaluation to data privacy and security and bias management.
- Retrieval Augmented Generation architecture: For efficient Gen AI-based co-pilots, the embeddings/vector storage databases, their hosting environment (inhouse/ dedicated/ shared), the type of chunking strategies supported, and the type of search (cosine/ semantic /hybrid) assessment is required for the best outcomes as per the use case.
AI Service / Co-pilot tool Attributes: The AI model and the Co-Pilot tools attributes significantly impact the adoption of the solution and the below to be considered:
- Computational resources: Computation has a huge cost impact, so evaluation is required for lighter framework-based solutions.
- Model Attribute: Critical evaluation criteria for attributes are model training requirements, Input token size limitation, specialization (like summarization, text-to-code, etc.), seeding/grounding capability, availability in different regions, self-hosted or model as a service, model maturity level, and inference time.
- Deployment platforms: The environments where AI models are deployed and made operational are important. Having a sandbox environment for quick proofs of concept, synthetic data for training purposes, and developing a library of reusable AI components enables faster prototyping and go-to-market capability.
- Regulatory compliance: Adhering to laws and regulations governing AI usage.
Performance metrics: It is critical to assess the response regarding consistency, accuracy, ethics, and compliance against the benchmark.
- Scalability: The co-pilot’s flexibility to adapt to the specific use case and scale are important parameters for an enterprise-level release.
- Explainability: Explainability of the outcomes generated by the model, interpreting the behavior of the models in a human-understandable manner ensures transparency and enhances reliability.
- Integration capabilities: How easily the co-pilot can integrate with existing systems determines it scalability across the enterprise.
- Compatibility with different data and file types: The capability and the maturity of the Co-Pilot to work with different data and file types are important to consider against the business use case. The glide path of maturity of the co-pilot is also to be considered to measure fitment with future expectations.
- Response Time: Maintaining a critical response time while maintaining accuracy is crucial for the adoption of the solution. Response time management through load balancing and distributed regions greatly impacts user experience.
- Ethical Principles: Ensuring Co-pilots are fair and do not perpetuate biases.
- Regulatory Compliance: Measures, guardrails and controls of adhering to laws and regulations governing AI usage.
Investment Roadmap / Cost of Ownership:
- Cost of operations: The operating cost to run the co-pilot in production, entailing licensing model, compute requirement, cloud resource utilization, and skillset requirement for support and maintenance significantly contribute to the deployment strategy.
- Business Value: Align investment with expected outcomes and meticulously measure how the AI capability impacts business metrics and processes.
Copilots will evolve with time and become smarter and smarter only if the learnings on these above categories are carefully evaluated and adopted and the business value gained would be incredible.
Title picture: freepik.com