Business Impact creation through Data Sciences: An approach
3AI February 4, 2021
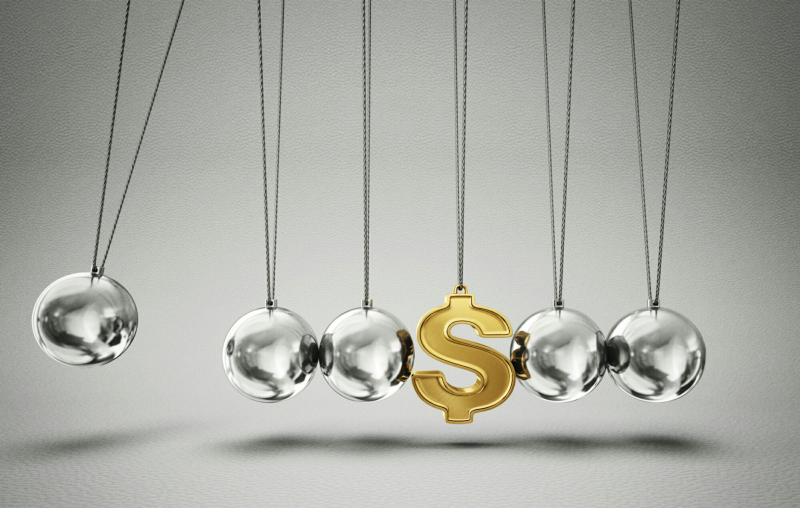
As per a recent study , on an average an individual organization will spend about $7.4M on data-related initiatives over the next twelve months , with enterprises investing $13.8M, and small & medium businesses (SMBs) investing $1.6M. 80% of enterprises and 63% of small & medium businesses (SMBs) already have deployed or are planning to deploy data sciences projects in the next twelve months.
36% of enterprises expect their IT budget allocations for data-driven initiatives to increase in 2015, 41% anticipate budget levels will remain at current levels and 21% aren’t sure. Only 3% say data-driven and big data-related project funding will decrease.
Data sciences continues to accelerate as the most preferred solution for gaining greater business insight and value from data, with this category increasing in importance 55% from 2014 survey results. In enterprises, data sciences (65%), visual dashboards (47%), data mining (43%), data warehousing (40%) and data quality (39%) are the five most preferred solutions. In financial services and manufacturing companies, the shift away from pre-built dashboards with common metrics and key performance indicators (KPIs) to the flexibility of defining their own data models in metrics is the future. Dashboards in financial institutions need to have the flexibility of quickly integrating entire new metrics and KPIs as their business models change. For manufacturers, the need for interpreting shop floor data to financial results is what’s driving data analysis and dashboards in the many manufacturing industries adopting analytics today.
There has been an ongoing considerable shift in the budgets, reallocated to data-driven activities like advanced analytics, majorly to focus on creating business impacts in the following sphere:
Innovation — Foster New Thinking Based on Data Sciences
Without data scientists and their knowledge, many issues surrounding the digital business age will remain unresolved — possibly even untouched. Data scientists frame complex business problems as machine-learning or operations research problems. Data scientists know which new information sources should be collected or acquired from external sources, to solve old burning business issues in radically new ways.
There are many more examples of disruptive projects and new “business moments” made possible through data:
- In the 1990s, Google achieved its incredible success by using a previously untapped data
- Also in the mid-1990s, Amazon started one of the earliest recommendation services (“here are four other items that customers buying this product also bought”), which became one of the most prominent and lucrative data science projects in history. Rumor has it that 15% to 20% of Amazon’s retail business is due to this simple product recommendation. In fact, it became a desirable feature, with customers wanting to explore related items for any given product.
- UPS On-Road Integrated Optimization and Navigation (ORION) revamped route optimization using many new data sources. It has enabled UPS to significantly improve its routing schedules, saving hundreds of millions of dollars per year while improving customer service.
Business Understanding — Explore Yet Unknown Patterns in Data
Data scientists must engage with data expeditions, especially when there is no clear objective other than to explore the data for insights and tidbits. Such expeditions are a form of inductive thinking or inductive reasoning— an example of “letting the data speak.” The process can be tactical and ad hoc. Alternatively, it can be part of a more systematic practice in which you give the data science team a data dump for diving into and exploring. The lab then looks for anomalies, seeking something new. The most basic techniques are:
- Looking at the basic shape of the data by examining univariate and bivariate distributions, correlation coefficients and all sorts of visualizations.
- Drilling deeper into the shape of the data using more advanced techniques. These might include cluster and factor analysis, anomaly detection, regression, decision trees, Monte Carlo simulation and link analysis.
The objective is always to:
- Discover which events are drivers — or inhibitors — of other events, or of good or bad outcomes (such as reducing equipment failure and increasing customer satisfaction).
- Gain an understanding of events that could be new customer touchpoints or engagement points. Such information could be used to foster data-based innovation.
Prototyping — Challenge the Status Quo With Radical New Solutions
Data sciences and especially machine learning excel in solving complex, data-rich business problems where traditional approaches, such as human judgment and exact solutions, either increasingly fail or deliver inferior solutions (see “Machine Learning Drives Digital Business”). Data science methods have been proven to often deliver superior results, when the space of critical variables is highly dimensional and very noisy. Hundreds of new business problems exist that data science teams could tackle. Companies are already using data science teams for tasks such as:
- Improving product categorization. Many large online retailers realize that their product classification may have errors or not fit the way customers think about products or want to access them. Data science teams are seeking to improve product categorization by using all available features. These include: look, shape, purpose, codes (such as European Article Numbering and North American Industry Classification System codes), product text descriptions and user-generated tags.
- Predicting more accurately which passengers who buy airline tickets will fail to arrive for their flights. More accurate predictions enable airlines to oversell their planes. This minimizes potential lost revenue from empty seats while reducing the risk of passengers arriving to find that there is no seat available for them.
Refinement — Continuously Improve Existing In-Production Solutions
Most data scientists in the industry work in the production part of the business. In such areas, established models are already “in production.” For example:
- Banks, retailers, telcos and insurance companies are constantly refining their existing customer segmentation, in order to gain a better understanding of customer profitability and customer behavior and engagement optimization.
- Retailers keep recalibrating propensity-to-buy models while online retailers are constantly Improving and updating price elasticity prediction, in order to optimize their dynamic pricing.
- Financial services providers are continuously working to improve their risk models — the more accurate their assessment of risk, the better their chances of profitability.
In all these use cases, organizations must constantly improve advanced analytics because:
- New data keeps arriving, simply due to new products being created all the time.
- Customer behavior changes not only by season, but also year after year (through competition, the zeitgeist and an ever-changing marketplace).
- Customer touchpoints change each year, and sometimes even more quickly.
- New customer segmentation strategies can lead to better results — and require many existing models to be adjusted.
Firefighting — Identify the Drivers of Certain Upcoming Situations
Sometimes it may be almost impossible to avoid a crisis because insights into issues that may cause problems can be so well hidden. In such cases, use your data science team to help resolve the crisis. This use is a variation of the data expedition use of data science teams. Many data science projects are triggered by crises. When you ask a data science team in this way, you already know the “symptom” of the crisis. For example:
- Customer complaints have suddenly risen
- Customer retention has fallen dramatically
- Quality defects have increased
- Profitability has dropped
This means that the data science team has to identify “only” the cause, which narrows the datasets it must scrutinize. Everything else in this use scenario is very similar to the work the lab does in data expeditions. As in big data expeditions, the lab does not know at the outset whether it can identify the cause of the problem. Indeed, it is possible that the lab may never be able to identify the cause.
Basic data discovery/self-service business intelligence can often help. However, a deeper dive by a data science team can extract more from the data about what is really happening. For example:
- Manufacturers worldwide are looking into the causes of quality fluctuations by combining “what if” analysis with sensitivity analysis or inversion of predictive models.
- Technical support operations are trying to understand the drivers of maintenance costs. It is known that certain customer segments are more difficult to deal with than others. Factoring these risks into pricing can be crucial and is a well-established practice in the insurance industry.
- Online retailers are investigating the reasons why customers return purchased goods.