How to blend Design Thinking with Data Sciences
3AI January 16, 2021
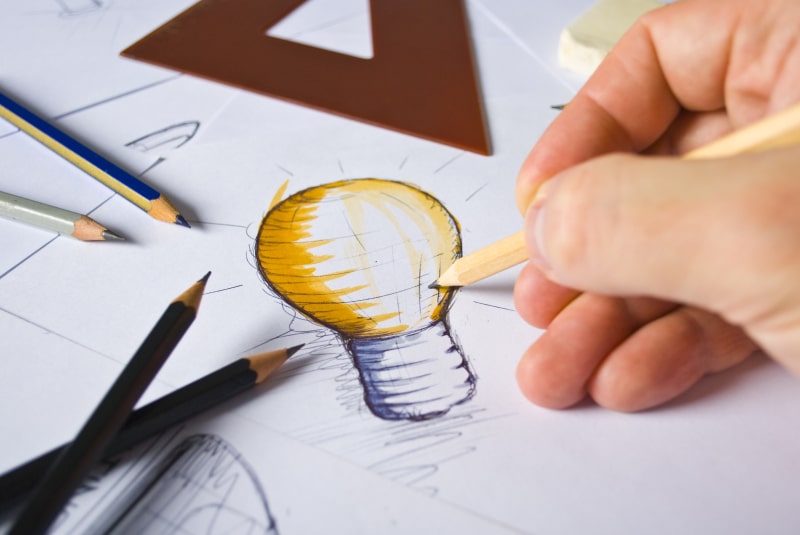
Organizations are operating in an increasingly complex environment. They extend across traditional boundaries in both market reach and operational footprint. Operations often involve messy and complicated human interactions either through customers, partners, and/or employees. To further complicate matters, organizations are tapping into increasingly sophisticated technology capable of capturing oceans of data with varying degrees of structure and quality. Many organizations have turned to data science to tackle today’s complex organizational challenges. They still struggle, however, to capitalize on the power of data to address their most important business challenges. Therein lies the data science challenge. How does an organization focus the strength of its data and its science to create impactful organizational value?
Two key dimensions make it hard for organizations to appropriately focus their data and its insight analysis. First, they need to have a deep and innate understanding of their business. While this may seem obvious, the reality is that this type of understanding is often dispersed among organizational leaders, business units, data scientists, individual employees, and customers. It requires an insatiable curiosity and institutional collaboration to discover. Without this level of understanding, the organization will not be able to identify and articulate the most pressing business issues, and there is no way to ensure that the organization’s analytical horsepower is cohesively focused on rich and relevant business questions. Second, the organizational value desired from data science is often found at the intersection of analytics and context. While data science can provide predictive and perspective analytics, insightful context can explain the answer of “why,” including “why does this matter.” Without the proper context, analytics will only go so far.
As a case, Data scientists who are centralized in one government agency’s organization are empowered to prioritize their own projects. Often the data scientists use financial return as the primary criterion to prioritize their efforts. This essentially means that projects focused on other strategic objectives, such as improving customer experiences or decreasing propensity for operational errors, are deprioritized. Those responsible for such outcomes must often design and plan solutions without the benefit of the analytical insight that data science can provide. The risk here is that data scientists may expend their tremendous talent on questions that only serve pockets of the enterprise, rather than delivering on the promise of data science that can drive the collective enterprise to the next level of performance.
Design Thinking Rises to the Data Sciences Challenge: Grounding and Amplifying the Analytics Method
Solving the data science challenge is about an organization’s ability to focus, embrace, and use data science practice to generate meaning and impact that can result in the next level of organizational performance. One way to do this is to inject the art of design thinking into an organization’s data science approach. Design thinking is a problem-solving and innovation methodology—a tool box of techniques born from the designer’s mindset. It emphasizes solving problems by starting with people (e.g., customers, employees, patients) rather than starting with technology or business positioning. In particular, design thinking can be a powerful complement to data science, given its natural ability to support the seamless shift between deductive and inductive reasoning. Design thinking follows a (i.e., context) and builds the institutional knowledge and critical buy-in necessary to ensure ongoing engagement and eventual scaling of capabilities and solutions. These techniques refocus data efforts into more meaningful and important questions that are both business focused and analytically meaty.
Embedding Design Thinking Into Data Sciences
Design thinking is both an end-to-end process and a toolbox from which to pull tools and techniques for modular application. As such, its integration with data science can take several forms and requires both experienced practitioners and sufficient training of data scientists and other stakeholders to achieve a shared mindset and language from which to collaborate. Because we believe it is such a powerful complement, we train our own data scientists and our clients in these techniques so that they can get the most organizational value possible.
Blend Design Research Into Data Sciences
One of the key aspects of design thinking is looking for the hidden meaning or goals of the customer, employee, partner, or patient, etc. It’s not enough to identify and understand a customer’s need—organizations need to dig deeper. Establishing a design research capability and conducting research in sequence with quantitative methods of research (e.g., surveys, multivariate testing) helps to generate a more complete picture of not just what’s happening, but why. This can propel data science organizations in new directions through new levels of insight into problems that have interactions among humans (customers, employees, partners, etc.). The result can be a more fulfilling analytical answer for all parties involved.
Host Collaborative Problem Reframing Workshops
These can bring together data scientists, business owners, and even customers (where appropriate) to explore and discover the hidden roots of business challenges and reframe problems into more meaningful questions. Reframing workshops are designed to challenge inherent assumptions made during the analytical process, allowing the potential for breakthrough thinking and solution development. Greater value from data can be unleashed by following a progressive cycle of analytical testing and reframing to arrive at more promising (and elegant for that matter) analytical solutions. Greater collective understanding helps to design more insightful research questions, and when paired with the right analytical technique, increases the potential for generating notable business impact.
Use Structured Ideation To Think Big
Design thinking includes many techniques for triggering ideas, drawing on existing patterns, solutions, and concepts and reapplying them in novel ways. With our design thinking techniques, ideation moves from a critical linear process of idea-constraint-idea-constraint to a sequenced divergent process of generating a wealth of ideas before converging on the most promising. These techniques allow teams to turn insights from analysis into “so what” actions necessary to move toward organizational value.
RAPIDLY PROTOTYPE IN LOW-FIDELITY WAYS
Design thinking generates low-fidelity prototypes to demonstrate applicability and test ideas quickly and cheaply before making significant investments. These prototypes span mock-ups, illustrations, paper-based interfaces, narratives, and other techniques that are quick and low cost to produce and easy to test with customers and stakeholders in near-real time. As feedback is gathered, teams can build smarter implementation roadmaps. For example, one technique we practice is a “cupcake road mapping” approach in which solutions are planned in delightful portions with increasing complexity added only when a solution proves worthwhile. This allows organizations to dedicate resources to only the most viable solutions that can make a difference.